Node representation method based on time sequence diagram neural network and incremental learning method
A neural network and sequence graph technology, applied in the field of incremental learning, can solve problems such as the inability to learn rich information of nodes, the inability to store a large amount of graph snapshot data, and increase
- Summary
- Abstract
- Description
- Claims
- Application Information
AI Technical Summary
Problems solved by technology
Method used
Image
Examples
Embodiment Construction
[0036] In order to facilitate the understanding of the present application, the present application will be described more fully below with reference to the relevant drawings. Preferred embodiments of the application are shown in the accompanying drawings. However, the present application can be embodied in many different forms and is not limited to the embodiments described herein. On the contrary, the purpose of providing these embodiments is to make the disclosure of the application more thorough and comprehensive.
[0037] In the node representation method and the incremental learning method based on the sequence diagram neural network provided by the present invention, for the processing of the sequence diagram, such as figure 1 As shown, first, preprocessing operations are performed on each sequence graph snapshot, including feature dimensionality reduction and graph pooling operations. Next, use each GCN model with a dual attention mechanism in the dual attention netw...
PUM
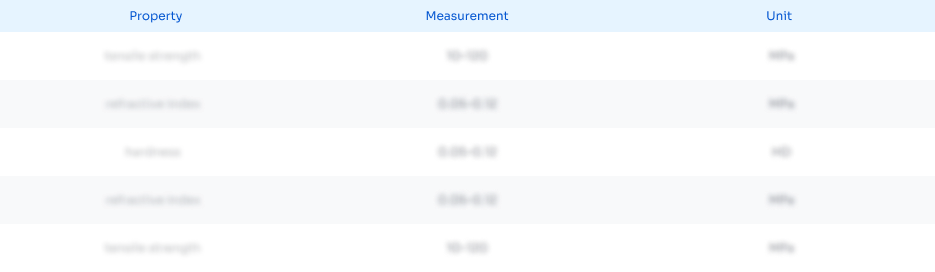
Abstract
Description
Claims
Application Information
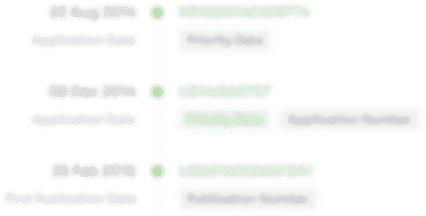
- R&D Engineer
- R&D Manager
- IP Professional
- Industry Leading Data Capabilities
- Powerful AI technology
- Patent DNA Extraction
Browse by: Latest US Patents, China's latest patents, Technical Efficacy Thesaurus, Application Domain, Technology Topic, Popular Technical Reports.
© 2024 PatSnap. All rights reserved.Legal|Privacy policy|Modern Slavery Act Transparency Statement|Sitemap|About US| Contact US: help@patsnap.com