Construction method of intelligent identification missing prevention system for breast ultrasound image lesions
An ultrasonic image and intelligent recognition technology, applied in image analysis, image data processing, instruments, etc., can solve problems such as delaying patient treatment timing and high missed diagnosis rate, and achieve the effects of reducing the cost of viewing films, robust and stable models, and improving efficiency
- Summary
- Abstract
- Description
- Claims
- Application Information
AI Technical Summary
Problems solved by technology
Method used
Image
Examples
Embodiment 1
[0041] A method for constructing a system for intelligent identification of lesions in breast ultrasound images and an anti-missing judgment system, such as figure 1 As shown, including S1. Data preparation, S2. Deep neural network model design, S3. Missed diagnosis prevention data consistency design, S4. Missed diagnosis prevention model training, S5. Missed diagnosis prevention model test.
Embodiment 2
[0043] The difference between this embodiment and Embodiment 1 is that the data preparation includes the following steps: S1.1. Data labeling: mark the exact position of the breast cancer lesion in the color Doppler ultrasound image to obtain the label of the corresponding image, each The color ultrasound image corresponds to a label, 0 represents normal breast or benign tumor, and 1 represents malignant tumor.
[0044] S1.2. Data preprocessing: For each color Doppler ultrasound report, information other than the color Doppler ultrasound image is cut out to prevent the interference of extra information. Restretch or shrink each color Doppler image to the same size to fit the network input size.
[0045] S1.3. Data set division: Divide the preprocessed color Doppler ultrasound image data into a training set and a test set at a ratio of 4:1 to evaluate the prediction results of the model and the prediction performance of the test model.
Embodiment 3
[0047] The difference between this embodiment and Embodiment 1 is that the neural network mainly consists of three parts: an input layer, a hidden layer, and an output layer. The hidden layer is composed of multiple neurons connected to each other, and various nonlinear transformations are used to fit the nonlinear relationship between the data, so as to achieve the function of predicting the image category. In the present invention, the hidden layer is composed of multiple layers of neurons to form a deep neural network, so as to learn deeper features to obtain the mapping relationship between input data and its features, making the model more stable and the prediction effect better.
[0048] The design of the deep neural network model includes the following steps: S2.1. Training data enhancement: the color Doppler ultrasound image to be trained will be randomly rotated at an angle, randomly centered, randomly vertically flipped, and randomly horizontally flipped to expand the...
PUM
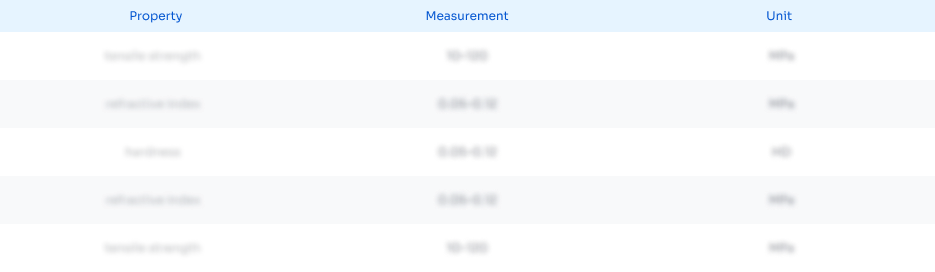
Abstract
Description
Claims
Application Information
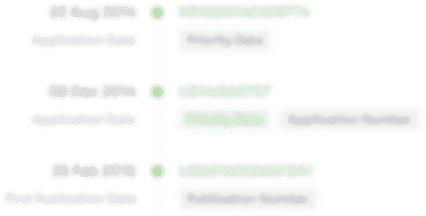
- R&D
- Intellectual Property
- Life Sciences
- Materials
- Tech Scout
- Unparalleled Data Quality
- Higher Quality Content
- 60% Fewer Hallucinations
Browse by: Latest US Patents, China's latest patents, Technical Efficacy Thesaurus, Application Domain, Technology Topic, Popular Technical Reports.
© 2025 PatSnap. All rights reserved.Legal|Privacy policy|Modern Slavery Act Transparency Statement|Sitemap|About US| Contact US: help@patsnap.com