Skeleton action recognition method based on graph convolution
An action recognition and skeleton technology, applied in the field of action recognition, can solve the problem that the local/global information and the corresponding relationship between specific actions and human body parts cannot be well considered, and achieve the effect of improving the accuracy.
- Summary
- Abstract
- Description
- Claims
- Application Information
AI Technical Summary
Problems solved by technology
Method used
Image
Examples
Embodiment
[0067] In order to verify the effectiveness of the scheme of the present invention, a simulation experiment was carried out on the public skeleton recognition task dataset NTU RGB+D based on the Pytorch deep learning platform. During the experiment, the method of the present invention follows two evaluation protocols of cross view and cross subject to determine training and test data, and then conducts training and testing of the deep graph convolutional network. When training the network, the training data is input to the deep graph convolutional network for forward propagation, and the classification probability of each sample for each action category is obtained, and then backpropagation is performed based on the cross-entropy loss to adjust the network parameters. After the training is completed, class prediction is performed on the sample to be tested based on the network, that is, the test sample is input into the trained deep image convolutional network, and the classifi...
PUM
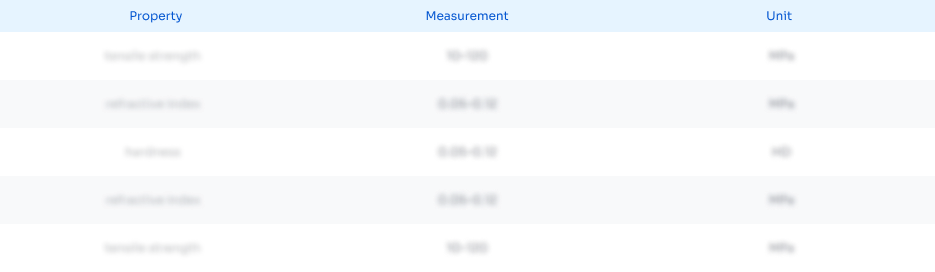
Abstract
Description
Claims
Application Information
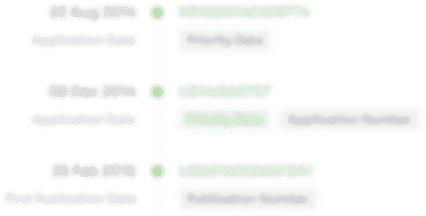
- R&D Engineer
- R&D Manager
- IP Professional
- Industry Leading Data Capabilities
- Powerful AI technology
- Patent DNA Extraction
Browse by: Latest US Patents, China's latest patents, Technical Efficacy Thesaurus, Application Domain, Technology Topic, Popular Technical Reports.
© 2024 PatSnap. All rights reserved.Legal|Privacy policy|Modern Slavery Act Transparency Statement|Sitemap|About US| Contact US: help@patsnap.com