Multi-head attention memory network for short text sentiment classification
A technology of emotion classification and attention, applied in text database clustering/classification, text database query, biological neural network model, etc., can solve problems such as difficulty in mining short text inline relations, effective coding of emotional semantic structure, etc.
- Summary
- Abstract
- Description
- Claims
- Application Information
AI Technical Summary
Problems solved by technology
Method used
Image
Examples
Embodiment 1
[0045] Such as figure 1 Shown, in embodiment 1, a kind of multi-head attention memory network that is used for short text emotion classification, network comprises multi-hop memory sub-network, and multi-hop memory sub-network comprises a plurality of sequentially connected independent computing modules, this implementation The example specifically includes two sequentially connected independent computing modules (hop), and the independent computing module includes sequentially connected first multi-head attention coding layer, first linear layer and output layer; the first multi-head attention coding layer According to the input historical information memory and original memory, the first linear layer linearizes the output of the first multi-head attention encoding layer, and the output layer superimposes the output of the first linear layer and the historical information memory to obtain a more accurate High-level abstract data representation. Wherein, the original memory i...
Embodiment 2
[0118] This embodiment has the same inventive concept as Embodiment 1. On the basis of Embodiment 1, a short text emotion classification method based on a multi-head attention memory network is provided. The method specifically includes:
[0119] S01: Obtain the word vector matrix of the short text, convert the word vector matrix into n-gram features and generate a new N-gram feature matrix, model the N-gram feature matrix, and mine the dependencies and relationships of each phrase in the text It hides the meaning and obtains the high-level feature representation of the input text;
[0120] S02: Perform abstract conversion of the n-gram feature sequence, perform multi-head attention calculation on the high-level feature representation, and finally perform linearization processing to obtain historical information memory;
[0121] S03: Perform multi-head attention calculations on historical information memory and N-gram features, and superimpose them after linear processing, and...
Embodiment 3
[0129] This embodiment provides a storage medium, which has the same inventive concept as Embodiment 2, and computer instructions are stored thereon, and when the computer instructions run, the multi-head attention memory network for short text emotion classification in Embodiment 2 is executed. step.
[0130] Based on this understanding, the technical solution of this embodiment is essentially or the part that contributes to the prior art or the part of the technical solution can be embodied in the form of a software product, and the computer software product is stored in a storage medium. Several instructions are included to make a computer device (which may be a personal computer, a server, or a network device, etc.) execute all or part of the steps of the methods in various embodiments of the present invention. The aforementioned storage medium includes: U disk, mobile hard disk, read-only memory (Read-Only Memory, ROM), random access memory (Random Access Memory, RAM), ma...
PUM
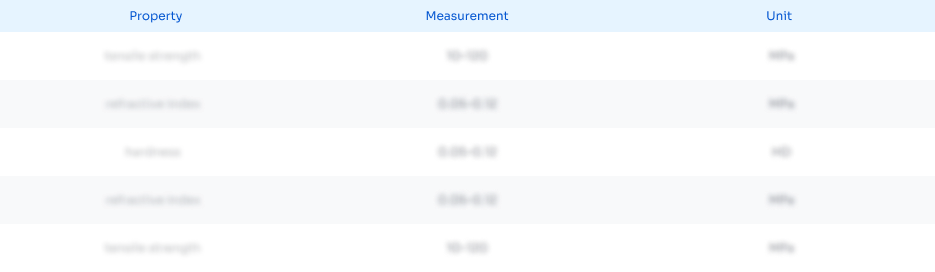
Abstract
Description
Claims
Application Information
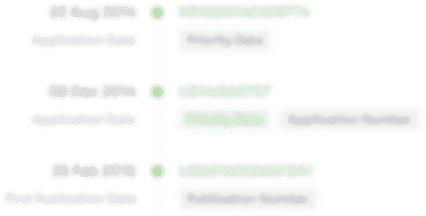
- R&D
- Intellectual Property
- Life Sciences
- Materials
- Tech Scout
- Unparalleled Data Quality
- Higher Quality Content
- 60% Fewer Hallucinations
Browse by: Latest US Patents, China's latest patents, Technical Efficacy Thesaurus, Application Domain, Technology Topic, Popular Technical Reports.
© 2025 PatSnap. All rights reserved.Legal|Privacy policy|Modern Slavery Act Transparency Statement|Sitemap|About US| Contact US: help@patsnap.com