Multi-agent deep reinforcement learning method, system and application
A reinforcement learning and multi-agent technology, applied in the field of multi-agent deep reinforcement learning, can solve the problems of long training time, slow neural network training speed, low learning efficiency, etc., and achieve high usability
- Summary
- Abstract
- Description
- Claims
- Application Information
AI Technical Summary
Problems solved by technology
Method used
Image
Examples
Embodiment Construction
[0039]In order to make the invention, according to the invention, the invention will be apparent from the accompanying drawings in the embodiment of the present invention, and the technical solutions in the embodiments of the present invention will be clearly described, and it is clear that the following The description described is merely the embodiment of the invention, and not all of the embodiments. Based on the embodiments of the present invention, there are all other embodiments obtained without making creative labor without making creative labor premises.
[0040]The present invention discloses a multi-intelligent depth strengthening method, including the following procedure:
[0041]1. Experience in partition cache
[0042]In a general multi-intelligent depth strengthening study, the smart body is implemented from one state transfer S to the next state S 'by performing a behavior A, and obtains the reward value R. Then, transfer this state to information EThe transfer information is ...
PUM
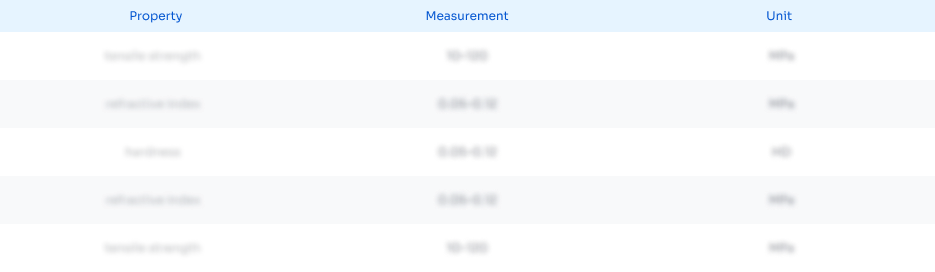
Abstract
Description
Claims
Application Information
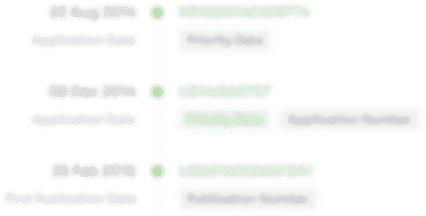
- R&D
- Intellectual Property
- Life Sciences
- Materials
- Tech Scout
- Unparalleled Data Quality
- Higher Quality Content
- 60% Fewer Hallucinations
Browse by: Latest US Patents, China's latest patents, Technical Efficacy Thesaurus, Application Domain, Technology Topic, Popular Technical Reports.
© 2025 PatSnap. All rights reserved.Legal|Privacy policy|Modern Slavery Act Transparency Statement|Sitemap|About US| Contact US: help@patsnap.com