Caenorhabditis elegans detection method based on multi-task deep neural network
A deep neural network and C. elegans technology, applied in the field of image processing, can solve problems such as large errors and low efficiency, and achieve the effect of improving accuracy and learning efficiency
- Summary
- Abstract
- Description
- Claims
- Application Information
AI Technical Summary
Problems solved by technology
Method used
Image
Examples
Embodiment Construction
[0030] The technical solutions in the embodiments of the present invention will be clearly and completely described below with reference to the accompanying drawings in the embodiments of the present invention. Obviously, the described embodiments are only a part of the embodiments of the present invention, rather than all the embodiments. Based on the embodiments of the present invention, all other embodiments obtained by those of ordinary skill in the art without creative efforts shall fall within the protection scope of the present invention.
[0031] A C. elegans detection method based on multi-task deep neural network, such as figure 1 As shown, the detection method includes the following steps:
[0032] Step 1: Obtain the original fluorescent image of C. elegans, such as figure 2 shown.
[0033] Step 2: The original fluorescent image is manually marked with the outline of the fluorescent point and the fluorescent brightness, and the image is expanded and preprocessed ...
PUM
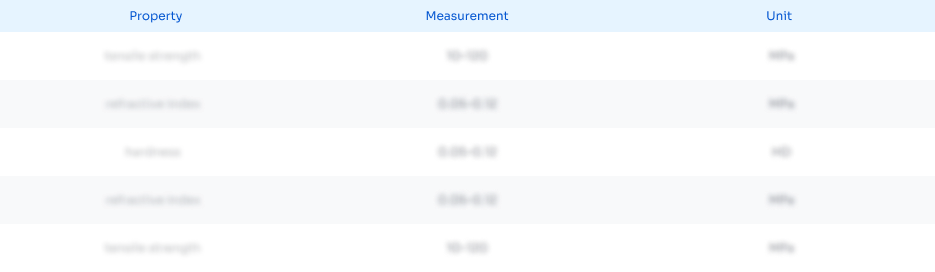
Abstract
Description
Claims
Application Information
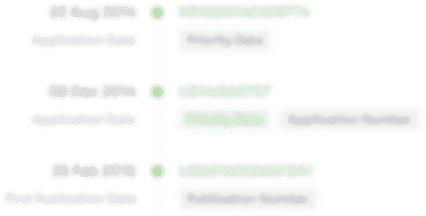
- R&D Engineer
- R&D Manager
- IP Professional
- Industry Leading Data Capabilities
- Powerful AI technology
- Patent DNA Extraction
Browse by: Latest US Patents, China's latest patents, Technical Efficacy Thesaurus, Application Domain, Technology Topic.
© 2024 PatSnap. All rights reserved.Legal|Privacy policy|Modern Slavery Act Transparency Statement|Sitemap