Software reviewer mixed recommendation method based on deep learning and multi-Agent optimization
A deep learning and hybrid recommendation technology, applied in neural learning methods, special data processing applications, instruments, etc., can solve the problems of mining developers and PR high-level feature information, without considering massive data and label feature dimensions, etc., to improve The effects of recommending accuracy, improving accuracy, and improving efficiency
- Summary
- Abstract
- Description
- Claims
- Application Information
AI Technical Summary
Problems solved by technology
Method used
Image
Examples
Embodiment approach
[0027] Such as figure 1As shown, the low-level feature processing part divides features into discrete features and continuous features. Discrete features are mapped to low-dimensional feature vectors through vector embedding, and are concatenated with continuous features to obtain new feature vectors, which is convenient for subsequent recommendation calculations. . Usually, the sample features obtained in the recommendation system are low-level features. These features include continuous features and discrete features. Continuous features correspond to real-valued data and do not need to be re-encoded. Therefore, the dimensions are limited and can be directly calculated. The type feature corresponds to the type feature. There is no real-valued data, and it needs to be encoded before it can be used. Usually, one-hot encoding is used. Therefore, if there are many possible value types for a certain type of feature, the one-hot encoding will be very long. Splicing many discrete ...
PUM
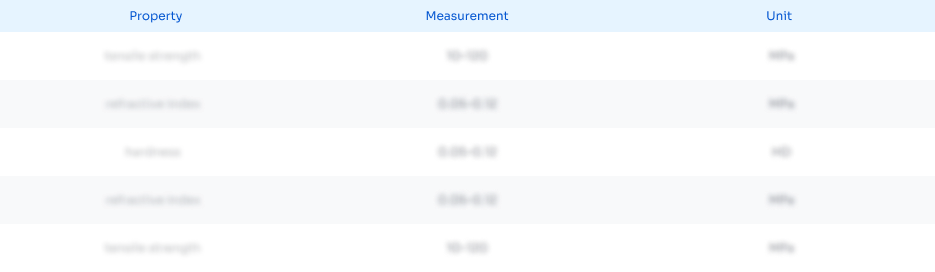
Abstract
Description
Claims
Application Information
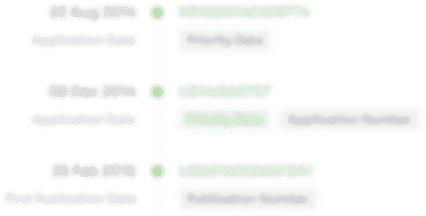
- R&D
- Intellectual Property
- Life Sciences
- Materials
- Tech Scout
- Unparalleled Data Quality
- Higher Quality Content
- 60% Fewer Hallucinations
Browse by: Latest US Patents, China's latest patents, Technical Efficacy Thesaurus, Application Domain, Technology Topic, Popular Technical Reports.
© 2025 PatSnap. All rights reserved.Legal|Privacy policy|Modern Slavery Act Transparency Statement|Sitemap|About US| Contact US: help@patsnap.com