Unsupervised feature selection method based on hidden space learning and popular constraint
A feature selection method and latent space learning technology, applied in complex mathematical operations, character and pattern recognition, instruments, etc., can solve problems such as performance impact
- Summary
- Abstract
- Description
- Claims
- Application Information
AI Technical Summary
Problems solved by technology
Method used
Image
Examples
Embodiment 1
[0081] This embodiment provides an unsupervised feature selection method based on latent space learning and popular constraints, such as figure 1 shown, including:
[0082] S11. Input the original data matrix to obtain the feature selection model;
[0083] S12. Embedding latent space learning into the feature selection model to obtain a feature selection model with latent space learning;
[0084] S13. Adding the graph Laplacian regularization term into the feature selection model with hidden space learning to obtain the objective function;
[0085] S14. Using an alternate iterative optimization strategy to solve the objective function;
[0086] S15. Sort each feature in the original matrix, and select the top k features to obtain an optimal feature subset.
[0087] This embodiment proposes a feature selection method based on latent latent space learning and graph-based manifold constraints (LRLMR). Specifically, traditional similarity graphs are constructed to characterize...
Embodiment 2
[0144] An unsupervised feature selection method based on latent space learning and popular constraints provided in this embodiment is different from Embodiment 1 in that:
[0145] This example is to fully verify the effectiveness of the LRLMR method of the present invention.
[0146] Test the performance of the LRLMR method on eight commonly used basic databases (ORL, warpPIE10P, orlraws10P, COIL20, Isolet, CLL_SUB_111, Prostate_GE, USPS), and compare it with the following nine currently popular unsupervised feature selection algorithms:
[0147] (1) Baseline: All original features are adopted.
[0148] (2) LS: Laplacian score feature selection, this method selects the features that best fit the Gaussian Laplacian matrix.
[0149] (3) MCFS: Multiple Clustering Feature Selection, which uses norms to normalize the feature selection process as a spectral information regression problem.
[0150] (4) RSR: Regularized self-representation feature selection, which uses norms to calc...
PUM
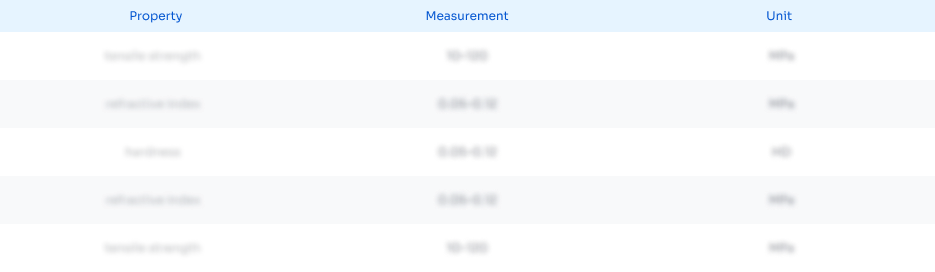
Abstract
Description
Claims
Application Information
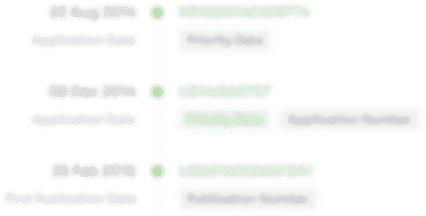
- R&D
- Intellectual Property
- Life Sciences
- Materials
- Tech Scout
- Unparalleled Data Quality
- Higher Quality Content
- 60% Fewer Hallucinations
Browse by: Latest US Patents, China's latest patents, Technical Efficacy Thesaurus, Application Domain, Technology Topic, Popular Technical Reports.
© 2025 PatSnap. All rights reserved.Legal|Privacy policy|Modern Slavery Act Transparency Statement|Sitemap|About US| Contact US: help@patsnap.com