Rod pumped well fault diagnosis method and system based on residual neural network
A neural network and pumping well technology, applied in the field of pumping well fault diagnosis based on residual neural network, can solve problems such as unsatisfactory, shortened model training time, few applications of pumping unit fault diagnosis, etc., to improve accuracy And the effect of good recall rate and convergence robustness
- Summary
- Abstract
- Description
- Claims
- Application Information
AI Technical Summary
Problems solved by technology
Method used
Image
Examples
Embodiment Construction
[0055] In order to make the technical means, creative features, goals and effects achieved by the present invention easy to understand, the present invention will be further elucidated below in conjunction with specific embodiments.
[0056] There are more than 20 downhole working conditions of oil wells in the oil field. When the number of working conditions increases, the shapes of the indicator diagrams representing different working conditions are very similar, and the difficulty of identification also increases. Therefore, an algorithm model with strong learning ability is required To learn the subtle differences between the indicator diagrams of different working conditions.
[0057] The dynamometer data collected at the oilfield production site is a sequence of suspension point load y(KN) and displacement x(m) in the stroke, with 120 or 240 data points, and the downhole working conditions can be judged according to the dynamometer diagram drawn. The pump depth and strok...
PUM
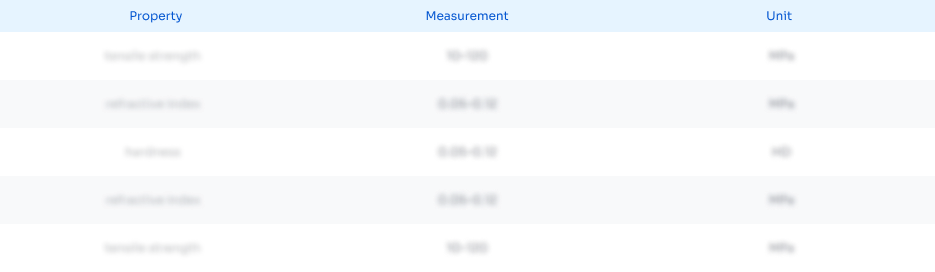
Abstract
Description
Claims
Application Information
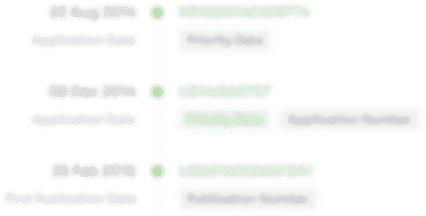
- Generate Ideas
- Intellectual Property
- Life Sciences
- Materials
- Tech Scout
- Unparalleled Data Quality
- Higher Quality Content
- 60% Fewer Hallucinations
Browse by: Latest US Patents, China's latest patents, Technical Efficacy Thesaurus, Application Domain, Technology Topic, Popular Technical Reports.
© 2025 PatSnap. All rights reserved.Legal|Privacy policy|Modern Slavery Act Transparency Statement|Sitemap|About US| Contact US: help@patsnap.com