Cell image detection method based on transfer learning
A technology of transfer learning and image detection, which is applied in the field of cell image detection based on transfer learning, can solve problems such as difficulty in labeling, size dependence, and lack of data sets, and achieve the effect of reducing difficulty in labeling, improving accuracy and recall rate
- Summary
- Abstract
- Description
- Claims
- Application Information
AI Technical Summary
Problems solved by technology
Method used
Image
Examples
Embodiment Construction
[0031] In the following description, the technical solutions in the embodiments of the present invention are clearly and completely described. Apparently, the described embodiments are only a part of the embodiments of the present invention, rather than all the embodiments. Based on the embodiments of the present invention, all other embodiments obtained by persons of ordinary skill in the art without making creative efforts belong to the protection scope of the present invention.
[0032] Embodiments of the present invention provide a cell image detection method based on migration learning, such as figure 1 As shown, the method includes the following steps:
[0033] A cell image detection method based on migration learning, characterized in that, comprising the steps of:
[0034] S1: Collect cell images, sample the low-resolution intensity images of the sample through the Fourier stack microscopic imaging system, obtain digital image data that can be directly processed by th...
PUM
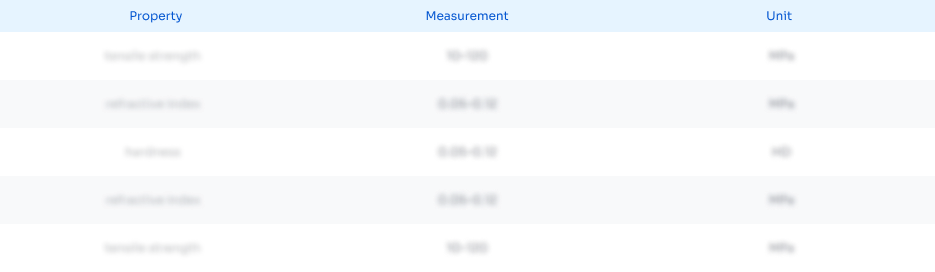
Abstract
Description
Claims
Application Information
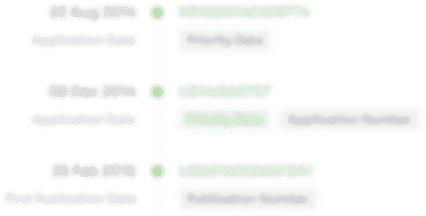
- R&D Engineer
- R&D Manager
- IP Professional
- Industry Leading Data Capabilities
- Powerful AI technology
- Patent DNA Extraction
Browse by: Latest US Patents, China's latest patents, Technical Efficacy Thesaurus, Application Domain, Technology Topic, Popular Technical Reports.
© 2024 PatSnap. All rights reserved.Legal|Privacy policy|Modern Slavery Act Transparency Statement|Sitemap|About US| Contact US: help@patsnap.com