Hyperspectral imaging method based on unsupervised network dedispersion blurring
A technology of hyperspectral imaging and hyperspectral imagery, which is applied in 2D image generation, image enhancement, image analysis, etc., and can solve problems such as taking a long time, limited model generalization ability, and insufficient model prediction accuracy to supervised learning. , to achieve good generalization ability and reduce the cost of data collection
- Summary
- Abstract
- Description
- Claims
- Application Information
AI Technical Summary
Problems solved by technology
Method used
Image
Examples
Embodiment Construction
[0028] In order to make the purpose, method and advantages of the present invention clearer, the implementation method of the present invention will be further described in detail below in conjunction with the accompanying drawings.
[0029] This embodiment provides a hyperspectral imaging method based on unsupervised network solution dispersion blur, see figure 1 , including:
[0030] S1: Collect RGB data with ambiguous dispersion.
[0031] The acquisition of dispersion RGB data can be realized through a dispersion component and an image sensor. Figure 5 (a) is the simulated dispersion RGB image D. The hyperspectral data S is a three-dimensional cube data with a size of y×x×c, where x and y represent the horizontal and vertical dimensions of the image in two-dimensional space respectively, c is the number of spectral channels, and the two-dimensional Data represent spectral signals in this band. Then the dispersion RGB image D can be expressed as:
[0032] D=ΩΦS
[003...
PUM
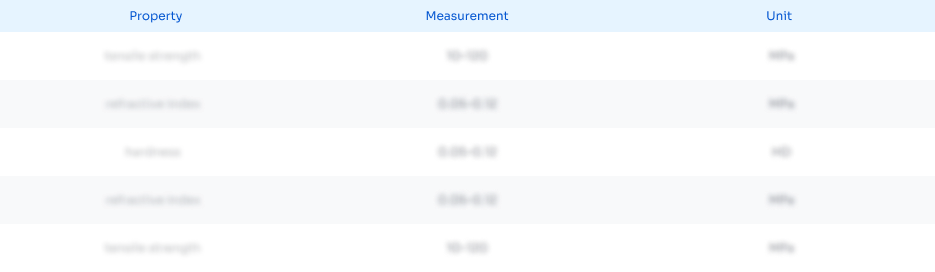
Abstract
Description
Claims
Application Information
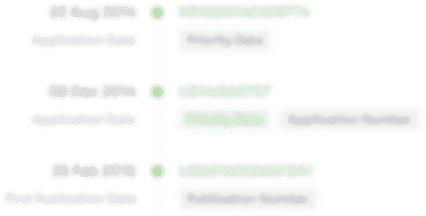
- R&D Engineer
- R&D Manager
- IP Professional
- Industry Leading Data Capabilities
- Powerful AI technology
- Patent DNA Extraction
Browse by: Latest US Patents, China's latest patents, Technical Efficacy Thesaurus, Application Domain, Technology Topic.
© 2024 PatSnap. All rights reserved.Legal|Privacy policy|Modern Slavery Act Transparency Statement|Sitemap