Urban main road speed prediction method based on big data and deep learning
A trunk road and speed prediction technology, applied in forecasting, data processing applications, traffic control systems of road vehicles, etc., can solve the problem of low accuracy of trunk road traffic speed, easy loss of correlation between different roads, and difficulty in extracting roads Speed and other issues, to achieve high prediction accuracy and reliability, less vehicle data features, and low cost
- Summary
- Abstract
- Description
- Claims
- Application Information
AI Technical Summary
Problems solved by technology
Method used
Image
Examples
Embodiment Construction
[0022] A kind of urban arterial road speed prediction method based on big data and deep learning of the present invention comprises the following steps:
[0023] Step 1. Call the Amap API interface to collect the static data of the city's main road map and create a static road set A. Specifically include the following steps:
[0024] Step 1.1, collect the names of the main roads in the city and the names of the driving roads with a large daily traffic volume.
[0025] Step 1.2. Call the Gaode map API interface to obtain the vector map data of the above roads, including: road latitude and longitude, vehicle driving direction, etc.
[0026] Step 1.3, if figure 1 As shown, any vector road Road j , according to the different driving directions of the vehicle, it is split into two independent roads Road j_1 and Road j_2 .
[0027] Step 1.4, if figure 1 As shown, the vector road (Road j_1 or Road j_2 ), so that each road is composed of several line segments connected end to...
PUM
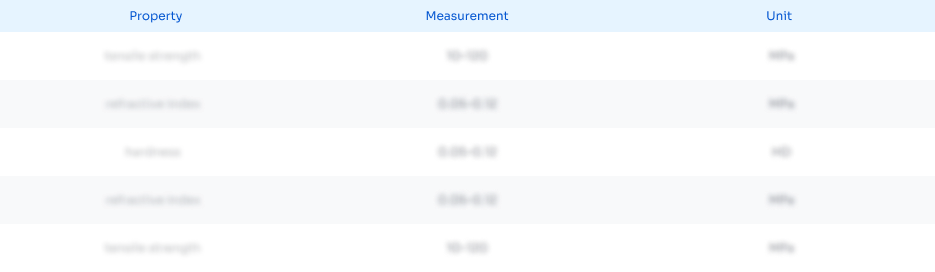
Abstract
Description
Claims
Application Information
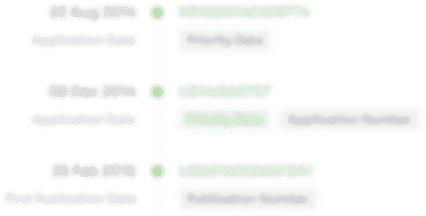
- R&D Engineer
- R&D Manager
- IP Professional
- Industry Leading Data Capabilities
- Powerful AI technology
- Patent DNA Extraction
Browse by: Latest US Patents, China's latest patents, Technical Efficacy Thesaurus, Application Domain, Technology Topic, Popular Technical Reports.
© 2024 PatSnap. All rights reserved.Legal|Privacy policy|Modern Slavery Act Transparency Statement|Sitemap|About US| Contact US: help@patsnap.com