Deep learning chip packaging crack defect detection method based on YOLO
A defect detection and chip packaging technology, which is applied in neural learning methods, image data processing, image enhancement, etc., can solve the problems of slow detection speed of YOLO network, difficulty in meeting industrial detection, and affecting detection effect, so as to improve network prediction speed, Good detection effect, reduce the effect of false prediction frequency
- Summary
- Abstract
- Description
- Claims
- Application Information
AI Technical Summary
Problems solved by technology
Method used
Image
Examples
Embodiment Construction
[0037] Embodiments of the present invention will be described in detail below. It should be emphasized that the following description is exemplary only, and is not intended to limit the scope of the invention and its application.
[0038] In order to correctly distinguish between good product pictures and crack pictures, the embodiment of the present invention provides a YOLO-based deep learning chip package crack defect detection method. The overall process of the method is as follows figure 1 As shown, it can be divided into seven steps: image acquisition, image annotation, data enhancement, model building, network training, network prediction and output post-processing.
[0039] The first step, image acquisition, collects industrial image data, and captures the image of the chip unit.
[0040] The second step, image labeling, uses an image labeling tool for labeling, and the labeling content is the defect category (ie crack) and the coordinates of the upper left and lower ...
PUM
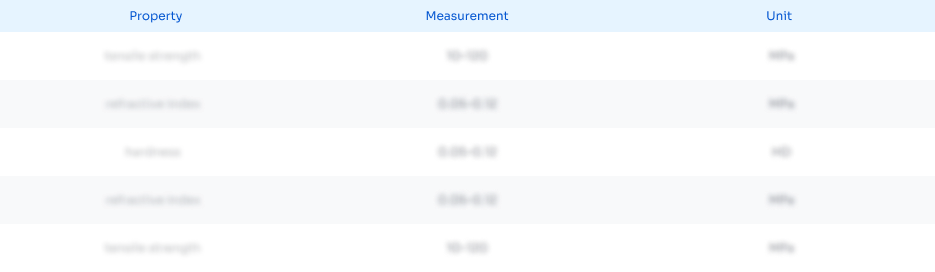
Abstract
Description
Claims
Application Information
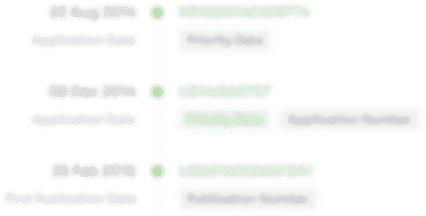
- R&D Engineer
- R&D Manager
- IP Professional
- Industry Leading Data Capabilities
- Powerful AI technology
- Patent DNA Extraction
Browse by: Latest US Patents, China's latest patents, Technical Efficacy Thesaurus, Application Domain, Technology Topic, Popular Technical Reports.
© 2024 PatSnap. All rights reserved.Legal|Privacy policy|Modern Slavery Act Transparency Statement|Sitemap|About US| Contact US: help@patsnap.com