Point cloud dynamic region graph convolution method, classification method and segmentation method
A technology of dynamic region and convolution method, applied in the field of computer vision, can solve problems such as insufficient storage performance accuracy, and achieve the effect of improving accuracy
- Summary
- Abstract
- Description
- Claims
- Application Information
AI Technical Summary
Problems solved by technology
Method used
Image
Examples
Embodiment Construction
[0022] The present invention will be further described in detail below in conjunction with the accompanying drawings.
[0023] Such as figure 1 As shown, the present invention discloses a method for convolution of point cloud dynamic area graphs, including:
[0024] S1. Acquire 3D point cloud data X, X={α 1 ,α 2 ,α 3 ,…,α i ,…,α n}, α i Represents the data of the i-th point, n represents the number of points in the 3D point cloud data, α i ={x i ,y i ,z i},x i 、y i and z i means α i three-dimensional coordinates;
[0025] S2. Perform two independent k-nearest neighbor operations on the three-dimensional point cloud data X to obtain two local feature maps y and z, and the k values of the two independent k-nearest neighbor operations are different;
[0026] Such as figure 2 As shown, it is the k-nearest neighbor graph of the local point cloud space, defining α j1 ,α j2 ,…,α jk for alpha i The k nearest neighbors of e ij is an edge feature, defined as e i...
PUM
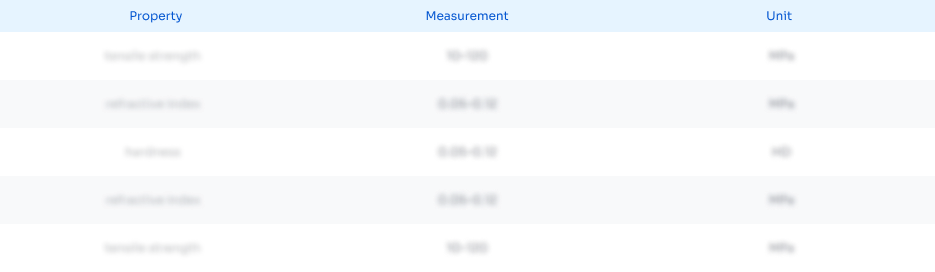
Abstract
Description
Claims
Application Information
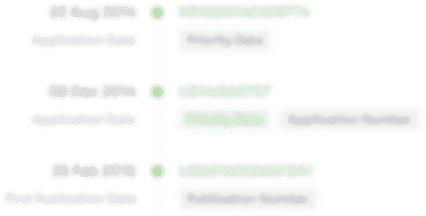
- R&D
- Intellectual Property
- Life Sciences
- Materials
- Tech Scout
- Unparalleled Data Quality
- Higher Quality Content
- 60% Fewer Hallucinations
Browse by: Latest US Patents, China's latest patents, Technical Efficacy Thesaurus, Application Domain, Technology Topic, Popular Technical Reports.
© 2025 PatSnap. All rights reserved.Legal|Privacy policy|Modern Slavery Act Transparency Statement|Sitemap|About US| Contact US: help@patsnap.com