Automatic indentation measurement method based on full convolutional neural network
A technology of convolutional neural network and measurement method, which is applied in the field of automatic indentation measurement based on full convolutional neural network, which can solve the problems that the complex indentation surface is difficult to function, the specific shape of the indentation cannot be extracted, and the material is inconvenient to break. , to achieve the effect of convenient actual deployment and application, convenient post-processing analysis, and wide application range
- Summary
- Abstract
- Description
- Claims
- Application Information
AI Technical Summary
Problems solved by technology
Method used
Image
Examples
Embodiment Construction
[0041] The present invention will be further described in detail below in conjunction with the accompanying drawings and specific embodiments.
[0042] An automatic indentation measurement method based on a fully convolutional neural network provided in this embodiment includes the following steps:
[0043] Step 1: Preprocessing the indentation image to obtain its binary image;
[0044] Step 2: Indentation image cropping: Find the maximum connected domain in the binary image, determine the bounding box of the maximum connected domain (as a reference for cropping); use the bounding box as a reference for cropping, expand the bounding box, and crop The expanded bounding box area on the binary image;
[0045] Step 3: Input the cropped image into the trained fully convolutional neural network, and output the binarized indentation pixel mask image (indentation pixels and non-indentation pixels are displayed as white and black, respectively), thus Accurate shape and position infor...
PUM
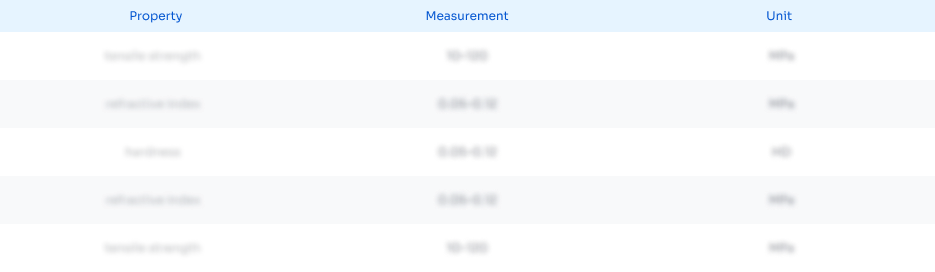
Abstract
Description
Claims
Application Information
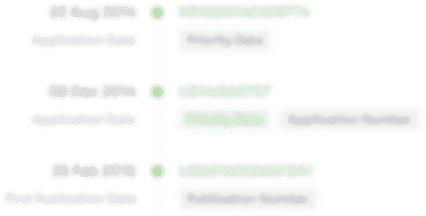
- R&D Engineer
- R&D Manager
- IP Professional
- Industry Leading Data Capabilities
- Powerful AI technology
- Patent DNA Extraction
Browse by: Latest US Patents, China's latest patents, Technical Efficacy Thesaurus, Application Domain, Technology Topic, Popular Technical Reports.
© 2024 PatSnap. All rights reserved.Legal|Privacy policy|Modern Slavery Act Transparency Statement|Sitemap|About US| Contact US: help@patsnap.com