Cross-category fault diagnosis method and system based on small sample learning, and storage medium
A technology of fault diagnosis and fault diagnosis model, applied in neural learning methods, character and pattern recognition, instruments, etc., can solve problems such as difficulty in fault diagnosis of mechanical components, and achieve the effect of clear classification boundary, guarantee feasibility, and guarantee accuracy
- Summary
- Abstract
- Description
- Claims
- Application Information
AI Technical Summary
Problems solved by technology
Method used
Image
Examples
Embodiment Construction
[0049] A kind of cross-category fault diagnosis method based on small sample learning proposed in this embodiment comprises the following steps:
[0050] H1. Build a fault diagnosis model: obtain the historical working data under the actual working conditions of part A, mark the signal category of the described historical working data of part A, and form marked data; construct a sample pair consisting of two described marked data, and Label whether the signal categories of two labeled data in the sample pair are the same or not, obtain a training set composed of multiple labeled sample pairs, and combine the training set for model training to obtain a fault diagnosis model. In this way, the fault diagnosis model is used to diagnose whether the two input fault data belong to the same signal category.
[0051] H2. Establish support set S: Obtain the historical working data of component B under the actual working conditions, select part from the historical working data of compone...
PUM
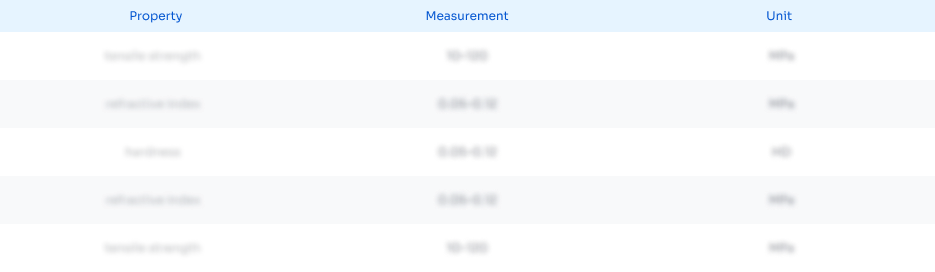
Abstract
Description
Claims
Application Information
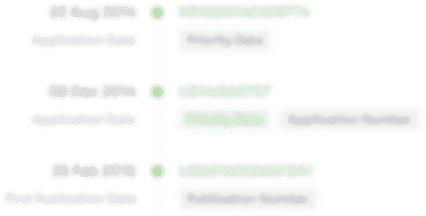
- R&D
- Intellectual Property
- Life Sciences
- Materials
- Tech Scout
- Unparalleled Data Quality
- Higher Quality Content
- 60% Fewer Hallucinations
Browse by: Latest US Patents, China's latest patents, Technical Efficacy Thesaurus, Application Domain, Technology Topic, Popular Technical Reports.
© 2025 PatSnap. All rights reserved.Legal|Privacy policy|Modern Slavery Act Transparency Statement|Sitemap|About US| Contact US: help@patsnap.com