Enhanced neurocognitive diagnostic model
A convolutional neural network and knowledge point technology, applied in the field of educational data mining, can solve problems such as not considering the importance of knowledge points, failing to capture the complex relationship between students and exercises, and affecting the diagnosis effect
- Summary
- Abstract
- Description
- Claims
- Application Information
AI Technical Summary
Problems solved by technology
Method used
Image
Examples
Embodiment Construction
[0009] See attached figure 1 As shown, the ENeuralCD model mainly consists of 4 parts:
[0010] 1. Vector representation of diagnostic factors:
[0011] In terms of students, this paper uses knowledge point proficiency vector α to represent students. α is obtained by multiplying the student one-hot encoded vector x with the trainable matrix A, namely
[0012] α=sigmoid(x×A)
[0013] where, α∈(0,1) 1×J ,x∈{0,1} 1×U ,A∈R U×J .
[0014] In terms of test questions, the knowledge point association vector p directly comes from the pre-given Q matrix:
[0015] p=z×Q
[0016] where p∈{0,1} 1×J , z∈{0,1} 1×V , Q∈{0,1} V×J , z is the one-hot encoded vector of the test item.
[0017] For other optional factors, use knowledge point difficulty vector β and test item discrimination γ. β=[β 1 ,β 1 ,...,β J ] indicates the difficulty of each knowledge point tested by the test questions. γ is used in test questions to distinguish students with high knowledge mastery from stude...
PUM
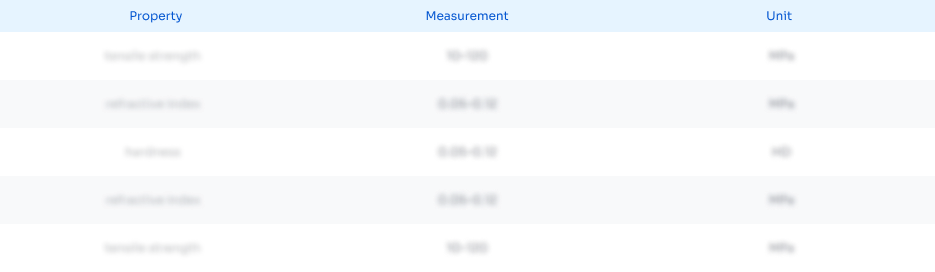
Abstract
Description
Claims
Application Information
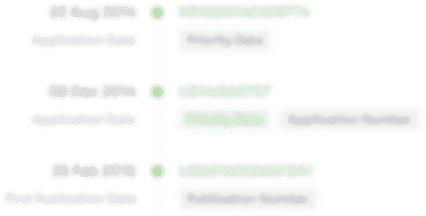
- R&D Engineer
- R&D Manager
- IP Professional
- Industry Leading Data Capabilities
- Powerful AI technology
- Patent DNA Extraction
Browse by: Latest US Patents, China's latest patents, Technical Efficacy Thesaurus, Application Domain, Technology Topic, Popular Technical Reports.
© 2024 PatSnap. All rights reserved.Legal|Privacy policy|Modern Slavery Act Transparency Statement|Sitemap|About US| Contact US: help@patsnap.com