ECG data classification method based on continuous deep learning
A data classification and deep learning technology, applied in medical science, diagnosis, diagnostic recording/measurement, etc., can solve problems such as poor classification effect, no display of computer analysis signal, and poor effect, so as to improve classification accuracy , to ensure the effect of classification accuracy
- Summary
- Abstract
- Description
- Claims
- Application Information
AI Technical Summary
Problems solved by technology
Method used
Image
Examples
Embodiment Construction
[0037] Embodiments of the invention are described in detail below, examples of which are illustrated in the accompanying drawings. The embodiments described below by referring to the figures are exemplary only for explaining the present invention and should not be construed as limiting the present invention.
[0038]The present invention utilizes the Elastic Weight Consolidation (EWC for short) method in Continuous Learning (CL for short) and Convolutional Neural Networks (CNN for short) to establish an automatic classification model for ECG data. In the present invention, the CNN model combined with the EWC method is used to classify two kinds of electrocardiogram data (ECG for short) with different sampling rates, so as to solve the problem of catastrophic forgetting in the training process and further improve the efficiency and accuracy of heartbeat classification. like figure 1 As shown, the specific process of the ECG data classification method based on continuous deep l...
PUM
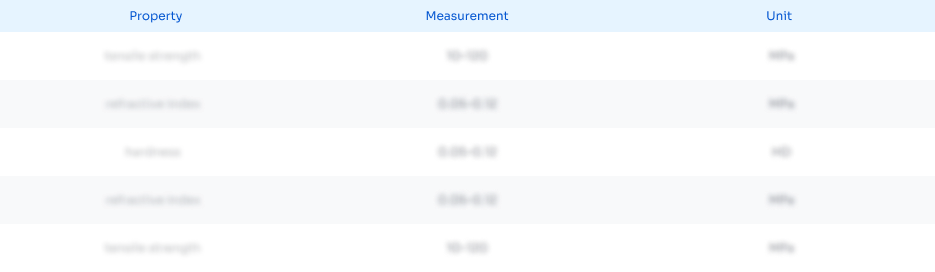
Abstract
Description
Claims
Application Information
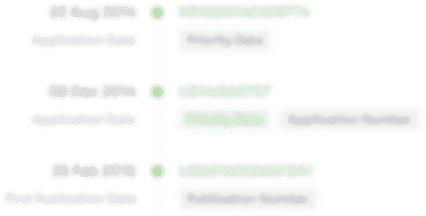
- R&D Engineer
- R&D Manager
- IP Professional
- Industry Leading Data Capabilities
- Powerful AI technology
- Patent DNA Extraction
Browse by: Latest US Patents, China's latest patents, Technical Efficacy Thesaurus, Application Domain, Technology Topic, Popular Technical Reports.
© 2024 PatSnap. All rights reserved.Legal|Privacy policy|Modern Slavery Act Transparency Statement|Sitemap|About US| Contact US: help@patsnap.com