A complex network link prediction method and system based on logical reasoning and graph convolution
A complex network and prediction method technology, applied in the field of complex network link prediction, can solve problems such as incomplete knowledge and low link prediction performance, and achieve the effect of high link efficiency and accurate relationship reasoning
- Summary
- Abstract
- Description
- Claims
- Application Information
AI Technical Summary
Problems solved by technology
Method used
Image
Examples
Embodiment Construction
[0067] In order to make those skilled in the art better understand the solutions of the present invention, the technical solutions in the embodiments of the present invention will be clearly and completely described below with reference to the accompanying drawings in the embodiments of the present invention. Obviously, the described embodiments are only Embodiments are part of the present invention, but not all embodiments. Based on the embodiments of the present invention, all other embodiments obtained by persons of ordinary skill in the art without creative efforts shall fall within the protection scope of the present invention.
[0068] In one embodiment, as figure 1 As shown, a complex network link prediction method based on logical reasoning and graph convolution is provided, which includes the following steps:
[0069] In step S10, an initial knowledge graph corresponding to the complex network is constructed, and a training set is obtained based on the initial knowle...
PUM
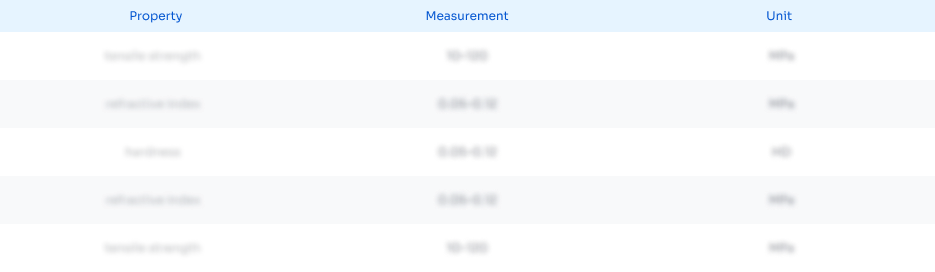
Abstract
Description
Claims
Application Information
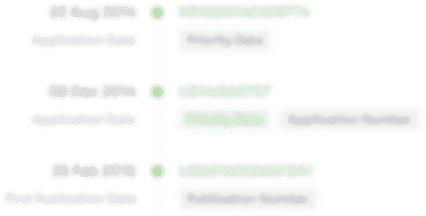
- R&D Engineer
- R&D Manager
- IP Professional
- Industry Leading Data Capabilities
- Powerful AI technology
- Patent DNA Extraction
Browse by: Latest US Patents, China's latest patents, Technical Efficacy Thesaurus, Application Domain, Technology Topic.
© 2024 PatSnap. All rights reserved.Legal|Privacy policy|Modern Slavery Act Transparency Statement|Sitemap