Noma system resource allocation method and storage medium based on optimized sample sampling
A system resource and allocation method technology, applied in the field of mobile communication and wireless network, can solve the problems of unlearned samples and low learning rate, achieve good sum rate performance, increase learning rate, and increase the effect of occurrence probability
- Summary
- Abstract
- Description
- Claims
- Application Information
AI Technical Summary
Problems solved by technology
Method used
Image
Examples
specific Embodiment approach 1
[0047] Specific implementation mode one: combine figure 1 with image 3 To describe this embodiment,
[0048] A kind of NOMA system resource allocation method based on optimized sample sampling described in this embodiment comprises the following steps:
[0049] (1) The base station obtains the channel state information of the user. The channel state information used in the present invention is channel gain.
[0050] (2) Use serial interference elimination technology to eliminate interference at the receiving end. For example, there are 3 users on the same channel, and the power allocated to the users is P 1 = 1W and P 2 = 2W and P 3 = 3W. At the receiving end, user 3 with the highest power is decoded first, and then the signal of user 3 is subtracted from the total mixed signal to obtain the mixed signal of user 1 and user 2. Then the last time the channel is weak (P 2 = 2W) user signal to perform serial interference cancellation technique, subtracting the signal of ...
specific Embodiment approach 2
[0069] This embodiment is a storage medium, and at least one instruction is stored in the storage medium, and the at least one instruction is loaded and executed by a processor to implement a NOMA system resource allocation method based on optimized sample sampling.
[0070] The present invention includes but is not limited to a storage medium, and may also be a device, the device includes a processor and a memory, the memory is a storage medium, which stores at least one instruction, and the at least one instruction is loaded by the processor And execute to realize a NOMA system resource allocation method based on optimal sample sampling.
PUM
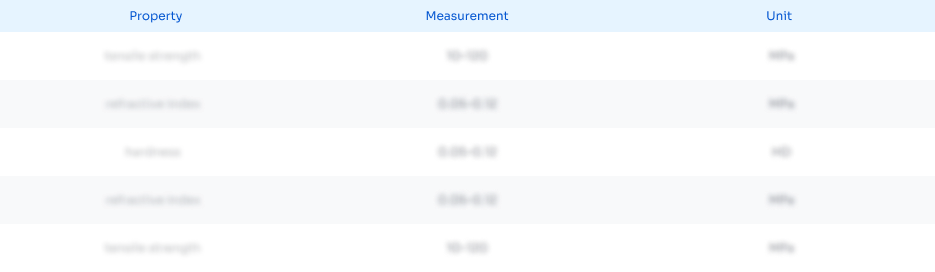
Abstract
Description
Claims
Application Information
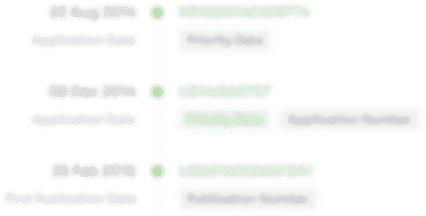
- R&D Engineer
- R&D Manager
- IP Professional
- Industry Leading Data Capabilities
- Powerful AI technology
- Patent DNA Extraction
Browse by: Latest US Patents, China's latest patents, Technical Efficacy Thesaurus, Application Domain, Technology Topic, Popular Technical Reports.
© 2024 PatSnap. All rights reserved.Legal|Privacy policy|Modern Slavery Act Transparency Statement|Sitemap|About US| Contact US: help@patsnap.com