A Deep Learning Neural Network Model System for Multimodal Image Synthesis
A neural network model and deep neural network technology, applied in the field of deep learning neural network model system, can solve the problems of lack of sCT image structure fidelity, and achieve the effect of high HU accuracy and good fidelity
- Summary
- Abstract
- Description
- Claims
- Application Information
AI Technical Summary
Problems solved by technology
Method used
Image
Examples
Embodiment Construction
[0023] Hereinafter, the present invention is described in more detail to facilitate understanding of the present invention.
[0024] Such as figure 1 with figure 2 As shown, the deep learning neural network model system for multimodal image synthesis described in the present invention includes a multi-resolution residual deep neural network formed by combining a residual deep neural network (RDNN) with a multi-resolution optimization strategy; The residual deep neural network described above includes 15 convolutional layers, and each convolutional layer can be expressed in the form of (k´k) conv, n, where k represents the size of the convolution kernel, and n represents the size of the convolution kernel number. In order to avoid network overfitting (overfitting), 7 dropout layers are added to the residual deep neural network (20% dropout rate); 7 batch normalization layers are used to normalize the input of the corresponding convolution kernel , thereby stabilizing the ne...
PUM
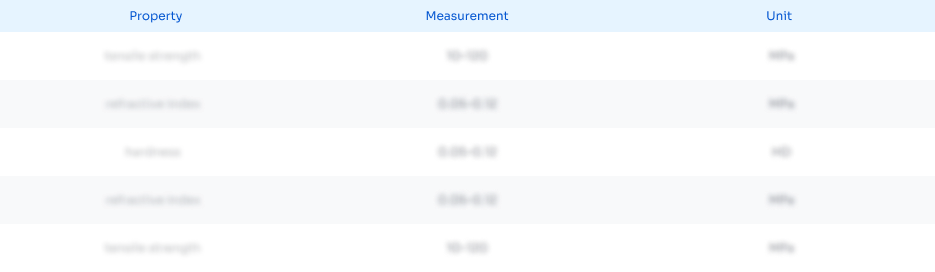
Abstract
Description
Claims
Application Information
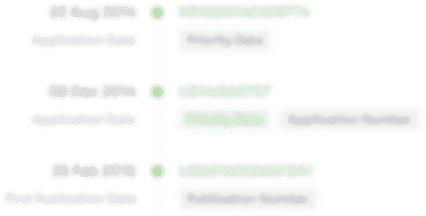
- R&D Engineer
- R&D Manager
- IP Professional
- Industry Leading Data Capabilities
- Powerful AI technology
- Patent DNA Extraction
Browse by: Latest US Patents, China's latest patents, Technical Efficacy Thesaurus, Application Domain, Technology Topic, Popular Technical Reports.
© 2024 PatSnap. All rights reserved.Legal|Privacy policy|Modern Slavery Act Transparency Statement|Sitemap|About US| Contact US: help@patsnap.com