Two-stage remote sensing target detection method based on target center point estimation
A target detection and center point technology, applied in neural learning methods, computing, computer components, etc., can solve problems such as damage to the accuracy of target detectors and unconsidered location distribution characteristics of remote sensing images.
- Summary
- Abstract
- Description
- Claims
- Application Information
AI Technical Summary
Problems solved by technology
Method used
Image
Examples
Embodiment
[0043] First, a two-stage target detection network (Centroid-Inference based Region Convolution Neural Network, CI-RCNN) based on target center point estimation is constructed according to the method of the present invention. Then obtain the training sample data, and use the sample data to train the network model. The sample data used in the embodiment is the remote sensing image data of Ningbo electric towers, including 228 training images and 76 test images, the image size is about 6000×6000 pixels, and all the electric tower labels have been manually inspected. We cut the original images in the training set into 1024×1024 pixel image blocks with a step size of 512 pixels, and input them into the network model for iterative training until the model converges to obtain the optimal weight file. After the model training is completed, the test remote sensing image to be detected is input into the trained network model for target detection, and the bounding box of the power tower...
PUM
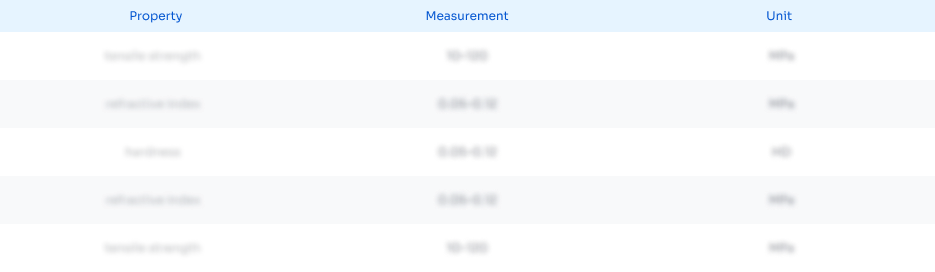
Abstract
Description
Claims
Application Information
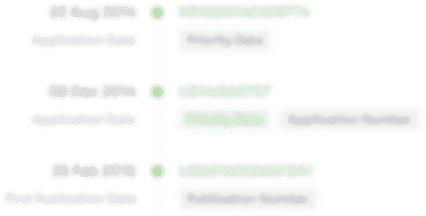
- Generate Ideas
- Intellectual Property
- Life Sciences
- Materials
- Tech Scout
- Unparalleled Data Quality
- Higher Quality Content
- 60% Fewer Hallucinations
Browse by: Latest US Patents, China's latest patents, Technical Efficacy Thesaurus, Application Domain, Technology Topic, Popular Technical Reports.
© 2025 PatSnap. All rights reserved.Legal|Privacy policy|Modern Slavery Act Transparency Statement|Sitemap|About US| Contact US: help@patsnap.com