R-CNN-based complex pavement crack identification method
A pavement crack and identification method technology, which is applied in the field of complex pavement crack identification, can solve the problems of poor robustness of the identification algorithm and high complexity of identification and measurement
- Summary
- Abstract
- Description
- Claims
- Application Information
AI Technical Summary
Problems solved by technology
Method used
Image
Examples
specific Embodiment approach 1
[0041] A kind of complex pavement crack recognition method based on R-CNN of this embodiment, such as figure 1 As shown, the method is realized through the following steps:
[0042] Step 1, pavement crack image preprocessing;
[0043] The performance of road pavement crack recognition based on deep learning depends on a large number of image data sets. However, in the process of road pavement crack recognition, there are problems such as insufficient training data, high data collection costs, and high labeling costs. It is very difficult to construct. The present invention adopts the migration learning method, utilizes the public dataset ImageNet with labels that has similar characteristics to road pavement cracks to pre-train Mask R-CNN, and collects the road pavement produced on the basis of pre-training. The crack data set optimizes the parameters of Mask R-CNN so that it can be used for crack identification on highway pavement.
[0044] In this way, it solves the problem...
specific Embodiment approach 2
[0055] The difference from the first embodiment is that in the R-CNN-based complex pavement crack recognition method of this embodiment, the step of pavement crack image preprocessing described in step 1 also includes the step of image expansion, specifically, for The data set is randomly mirrored, appropriate Gaussian noise is added, and the image is randomly rotated in the vertical direction to perform image enhancement. The enhancement of the data set makes up for the problem of insufficient data set samples to a certain extent, reduces the over-fitting phenomenon of the network, and can obtain a network with stronger generalization ability and better adapt to the application scenario.
specific Embodiment approach 3
[0057] Different from the second specific embodiment, in the present embodiment, a complex pavement crack recognition method based on R-CNN, the multi-layer neural network of the pavement crack recognition model described in step 2 is represented by assuming that the input of the first layer is x l , after several layers of neural network, the output is x L , then x L With the residual module, the output can be expressed as:
[0058]
[0059] In the formula, F(x i ,W i ) is the mapping output of a residual module; Wi is the weight of the i-th convolutional layer; bi is the bias of the i-th convolutional layer, assuming that the loss function output of the neural network is l, then after backpropagation, with the following results:
[0060]
[0061] It can be concluded from the above formula that when the residual module is added for backpropagation to update the weights, as the number of network layers deepens, there will be no phenomenon of gradient dispersion and g...
PUM
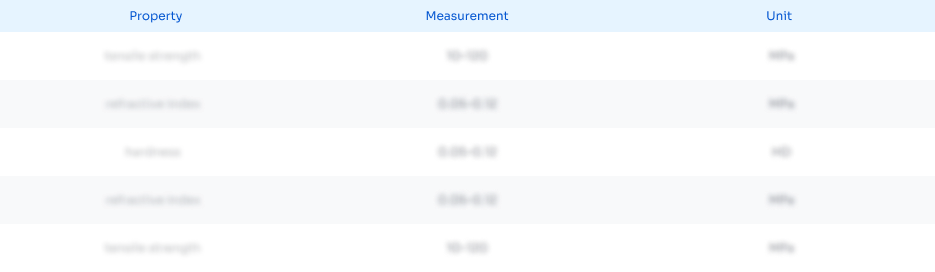
Abstract
Description
Claims
Application Information
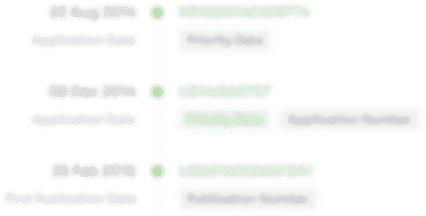
- R&D Engineer
- R&D Manager
- IP Professional
- Industry Leading Data Capabilities
- Powerful AI technology
- Patent DNA Extraction
Browse by: Latest US Patents, China's latest patents, Technical Efficacy Thesaurus, Application Domain, Technology Topic.
© 2024 PatSnap. All rights reserved.Legal|Privacy policy|Modern Slavery Act Transparency Statement|Sitemap