Low-illuminance image enhancement method and system based on image repair technology
An image enhancement and low-light technology, applied in the field of image processing, can solve the problems of halo at the edge, noise that cannot be removed, and strong noise that is difficult to enhance and restore, so as to achieve good visual effects and solve the effect of missing details
- Summary
- Abstract
- Description
- Claims
- Application Information
AI Technical Summary
Problems solved by technology
Method used
Image
Examples
Embodiment 1
[0065] In existing technical solutions, for example, although histogram equalization can adjust the brightness of the global image, it cannot remove noise and may reduce contrast. The algorithm based on the retinex theory decomposes the image into a reflection map and an illumination map, but it is prone to problems such as halos at the edges. In recent years, with the rapid development of deep learning, many algorithms based on deep learning have been proposed one after another. Although they can achieve good results in denoising and brightness adjustment, it is really difficult to recover the lack of details caused by noise.
[0066] For this reason, Embodiment 1 proposes a noise map-guided image repair network for low-illuminance image enhancement, and the technical solution is as follows:
[0067] Prepare the data set of low illumination image, used LOL data set and SYN data set among the present invention;
[0068] Preprocessing the data set, including pixel value normal...
Embodiment 2
[0081] On the basis of Embodiment 1, the applicant further researched and found that most existing low-illuminance image enhancement algorithms cannot remove noise well, and it is difficult to restore the problem of missing details caused by noise. Aiming at the problem of detail loss and difficulty in restoration, we propose a low-light image enhancement algorithm based on image inpainting technology.
[0082] The whole network structure diagram is as follows figure 1 As shown, it consists of a decomposition network and a restoration network in total. The decomposition network consists of three branches, which are responsible for generating reflection maps, illumination maps and noise maps, respectively. The restoration network is composed of Feature Enhancement Group (FEG) and Inpainting Module (InpaintingModule), such as image 3 shown. Each FEG is composed of 4 selection core enhancement modules (SKE), such as figure 2 shown. The decomposition network decomposes the ...
PUM
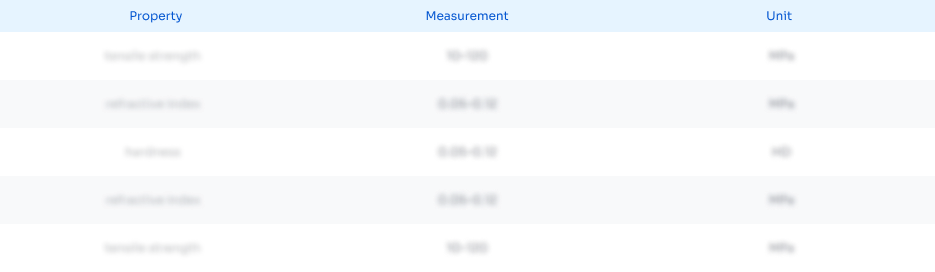
Abstract
Description
Claims
Application Information
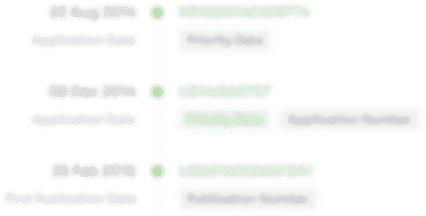
- R&D Engineer
- R&D Manager
- IP Professional
- Industry Leading Data Capabilities
- Powerful AI technology
- Patent DNA Extraction
Browse by: Latest US Patents, China's latest patents, Technical Efficacy Thesaurus, Application Domain, Technology Topic, Popular Technical Reports.
© 2024 PatSnap. All rights reserved.Legal|Privacy policy|Modern Slavery Act Transparency Statement|Sitemap|About US| Contact US: help@patsnap.com