Malicious code classification method based on deep residual network and mixed attention mechanism
A malicious code and classification method technology, applied in neural learning methods, biological neural network models, computer parts and other directions, can solve problems such as reducing the classification accuracy, single source of malicious code images, and increasing the difficulty of malicious code classification, etc. Classification accuracy and efficiency, the effect of efficient and accurate classification
- Summary
- Abstract
- Description
- Claims
- Application Information
AI Technical Summary
Problems solved by technology
Method used
Image
Examples
Embodiment Construction
[0029]The invention proposes a malicious code visual classification method based on a deep residual network and an attention mechanism. Among them, the malicious code visualization part uses the bytecode file and assembly file of the malware to visualize the malicious code as an RGBA image without additional calculation of the information entropy of the code, so as to make up for the single information source of the malicious code image, the unobvious image features and the amount of calculation. At the same time, the deep residual network is combined with the attention mechanism to build a malicious code classification model. The deep residual network can improve the classification accuracy while using short-circuit connections to alleviate the problem of gradient disappearance, accelerate model convergence, and improve The discriminative ability of the model is improved; and a mixed attention mechanism is used after each residual unit to extract more critical deep features fr...
PUM
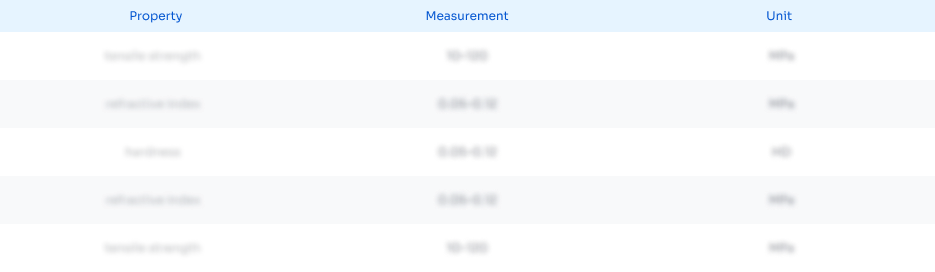
Abstract
Description
Claims
Application Information
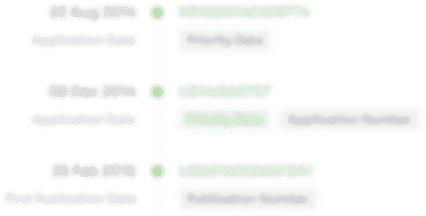
- R&D Engineer
- R&D Manager
- IP Professional
- Industry Leading Data Capabilities
- Powerful AI technology
- Patent DNA Extraction
Browse by: Latest US Patents, China's latest patents, Technical Efficacy Thesaurus, Application Domain, Technology Topic, Popular Technical Reports.
© 2024 PatSnap. All rights reserved.Legal|Privacy policy|Modern Slavery Act Transparency Statement|Sitemap|About US| Contact US: help@patsnap.com