Rotary machinery fault diagnosis method under complex working condition based on meta transfer learning
A technology of transfer learning and complex working conditions, applied in the field of energy manufacturing, can solve problems such as model performance degradation, achieve the effects of reducing selection restrictions, reducing demand, improving accuracy and generalization performance
- Summary
- Abstract
- Description
- Claims
- Application Information
AI Technical Summary
Problems solved by technology
Method used
Image
Examples
Embodiment Construction
[0022] In order to make the purpose, technical solution and advantages of the present invention clearer, the embodiments of the present invention will be further described below in conjunction with the accompanying drawings.
[0023] Please refer to figure 1 , the present invention provides a method for diagnosing faults of rotating machinery under complex working conditions based on meta-transfer learning, comprising the following steps:
[0024] S1. Collect the original sensor signals of mechanical equipment in different states, use the original data splicing method to convert the one-dimensional original signal into a two-dimensional time-frequency distribution image, and then obtain the corresponding three-channel time-frequency image through data expansion, as the fault diagnosis model in the present invention The input image dataset of ; please refer to figure 2 ,
[0025] The sample image is obtained by superimposing the original signal, assuming that the sequence X ...
PUM
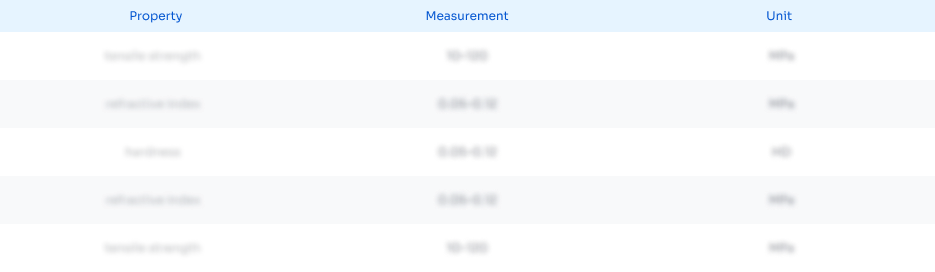
Abstract
Description
Claims
Application Information
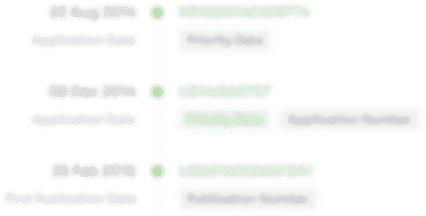
- Generate Ideas
- Intellectual Property
- Life Sciences
- Materials
- Tech Scout
- Unparalleled Data Quality
- Higher Quality Content
- 60% Fewer Hallucinations
Browse by: Latest US Patents, China's latest patents, Technical Efficacy Thesaurus, Application Domain, Technology Topic, Popular Technical Reports.
© 2025 PatSnap. All rights reserved.Legal|Privacy policy|Modern Slavery Act Transparency Statement|Sitemap|About US| Contact US: help@patsnap.com