Zero-sample visual classification method for cross-modal semantic enhancement generative adversarial network
A classification method and cross-modal technology, applied in biological neural network models, neural learning methods, computer components, etc., can solve problems such as easy collapse, deviation, and instability of generated information quality generated models, and achieve easy The effect of classifying and eliminating differences between modalities
- Summary
- Abstract
- Description
- Claims
- Application Information
AI Technical Summary
Problems solved by technology
Method used
Image
Examples
Embodiment
[0062] figure 1 It is a flowchart of a zero-shot visual classification method of a cross-modal semantically enhanced generative adversarial network of the present invention.
[0063] In this example, our model is based on generative adversarial networks (GANs) to solve the task of zero-shot learning by generating data of unseen classes. Traditional methods based on generative confrontation networks or other generative models directly generate visual features extracted by convolutional neural networks (CNN), and they often use the residual neural network (ResNet-101) pre-trained on the ImageNet dataset as the extracted features. architecture. However, such features themselves contain a large amount of label-independent information, so the generated features lack sufficient discrimination and increase the burden on the generation network. In addition, the instability of the generative model leads to poor quality of the generated visual features, and there is still a large gap ...
PUM
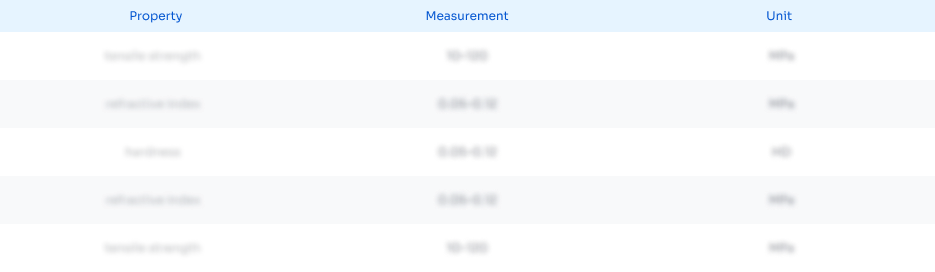
Abstract
Description
Claims
Application Information
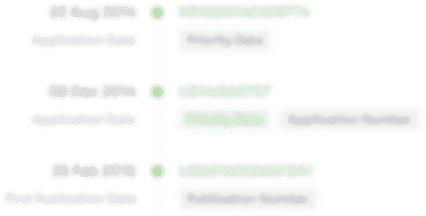
- R&D
- Intellectual Property
- Life Sciences
- Materials
- Tech Scout
- Unparalleled Data Quality
- Higher Quality Content
- 60% Fewer Hallucinations
Browse by: Latest US Patents, China's latest patents, Technical Efficacy Thesaurus, Application Domain, Technology Topic, Popular Technical Reports.
© 2025 PatSnap. All rights reserved.Legal|Privacy policy|Modern Slavery Act Transparency Statement|Sitemap|About US| Contact US: help@patsnap.com