State of charge estimation method for secondary battery, state of charge estimation system for secondary battery, and abnormality detection method for secondary battery
A secondary battery, state-of-charge technology, applied in anomaly detection systems, electronic equipment using neural networks, vehicles, processes, products or compositions, and machine fields using neural networks, can solve the problem of difficult to improve SOC estimation accuracy, accumulation, Problems such as the reduction of guessing accuracy
- Summary
- Abstract
- Description
- Claims
- Application Information
AI Technical Summary
Problems solved by technology
Method used
Image
Examples
Embodiment approach 1
[0066] In this embodiment, image 3 A procedure of performing a cycle test of a secondary battery as a reference, constructing a learning model based on the data, and estimating the capacity, and a procedure of detecting an abnormality using the learning model are shown.
[0067]First, a charge-discharge cycle test of a secondary battery as a reference was performed. (S1)
[0068] Collect the data obtained through the charge-discharge cycle test. (S2) In this data collection, various data are collected. For example, for CC time, CV time, temperature, discharge voltage, initial FCC (mAh), number of cycles, charge start voltage, voltage 1 second after charge start, voltage 2 seconds after charge start, 60 seconds after charge start The voltage after charging, the voltage after 120 seconds after charging, the voltage immediately after charging, the voltage that sleeps for 1 second after charging, the voltage that sleeps for 2 seconds after charging, the voltage that sleeps for...
Embodiment approach 2
[0104] In this embodiment, a comparison between Embodiment 1 and a comparative example different from Embodiment 1 will be described below with reference to FIG. 2 .
[0105] Figure 2A The result of obtaining the estimation error by changing the input data using the same learning model as in the first embodiment is shown.
[0106] Notice, Figure 2A and Figure 2B Input 3 shown with Figure 1A The inputs 3 shown are identical, which show the results under the same conditions.
[0107] in addition, Figure 2A and Figure 2B Input 5 shown is the result of using CC time and CV time, which is one of the inventions. In input 5, the average value is 5.9, and the minimum value is 3.2 compared to input 3, and the inference accuracy is lower than input 3.
[0108] in addition, Figure 2C The shown input 6, input 7, input 8, and input 9 are comparative examples, and the estimation errors of the comparative examples are all 10 (mAh) or more. The data of input 6 uses the chargin...
Embodiment approach 3
[0112] An example of a coin-type secondary battery will be described. Figure 9A It is an external view of a coin type (single layer flat type) secondary battery, Figure 9B is its cross-sectional view.
[0113] In the coin-type secondary battery 300 , a positive electrode can 301 serving as a positive terminal and a negative electrode can 302 serving as a negative terminal are insulated and sealed by a gasket 303 formed of polypropylene or the like. The positive electrode 304 is formed of a positive electrode current collector 305 and a positive electrode active material layer 306 provided in contact therewith. The negative electrode 307 is formed of a negative electrode current collector 308 and a negative electrode active material layer 309 provided in contact therewith.
[0114] Active material layers included in each of the positive electrode 304 and the negative electrode 307 used in the coin-type secondary battery 300 may be formed on only one surface.
[0115] As th...
PUM
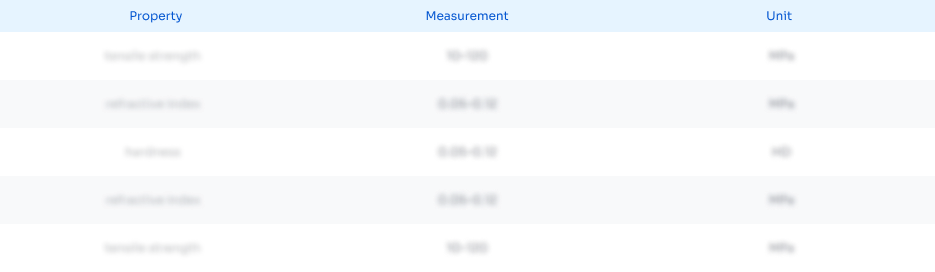
Abstract
Description
Claims
Application Information
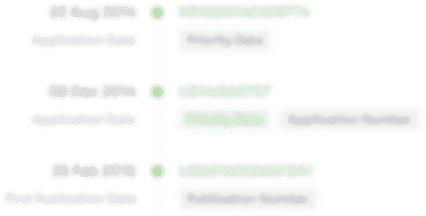
- R&D Engineer
- R&D Manager
- IP Professional
- Industry Leading Data Capabilities
- Powerful AI technology
- Patent DNA Extraction
Browse by: Latest US Patents, China's latest patents, Technical Efficacy Thesaurus, Application Domain, Technology Topic, Popular Technical Reports.
© 2024 PatSnap. All rights reserved.Legal|Privacy policy|Modern Slavery Act Transparency Statement|Sitemap|About US| Contact US: help@patsnap.com