Time-space domain obstacle detection method and system for complex road scene
An obstacle detection, space-time domain technology, applied in the field of space-time domain obstacle detection methods and systems, can solve the problems such as the inability to effectively detect the target, the difficulty of the model to give results, and the difficulty of target detection, so as to avoid position jumping, Accurate results and good effect of path planning
- Summary
- Abstract
- Description
- Claims
- Application Information
AI Technical Summary
Problems solved by technology
Method used
Image
Examples
Embodiment 1
[0052] Such as figure 1 and figure 2 As shown, this embodiment discloses a time-space domain obstacle detection method for complex road scenes, including the following steps:
[0053] S100: collecting point cloud data and detection results of historical frames, and preprocessing the point cloud data;
[0054] Point cloud data includes one frame of point cloud data at the current moment or / and multi-frame point cloud data of a certain period of time in the past; preprocessing of point cloud data includes but not limited to downsampling, noise removal, area filtering etc.
[0055] S200: Detect the preprocessed point cloud data using the deep learning method and the occupancy grid method respectively; the known type target information (known category detection results) is obtained through the detection by the deep learning method, and the occupancy grid method is used for detection. Detect unknown type target information (unknown type detection result);
[0056] The deep lea...
Embodiment 2
[0085] Such as Figure 4 As shown, this embodiment discloses a space-time domain obstacle detection system for complex road scenes, including a preprocessing module, a detection module, an association module and a fusion module;
[0086] The preprocessing module is used to collect point cloud data and detection results of historical frames, and preprocess the point cloud data;
[0087] The detection module is used to detect the preprocessed point cloud data using the deep learning method to obtain known type target information, and use the occupancy grid method to detect unknown type target information;
[0088] The association module is used to perform space-domain association fusion on the known type target and the unknown type target based on the known type target information and the unknown type target information to obtain the detection result of the current frame;
[0089] The fusion module is used to perform motion estimation on the detection result of the historical f...
PUM
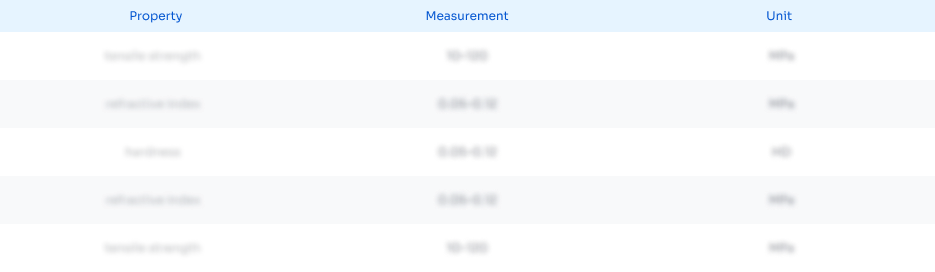
Abstract
Description
Claims
Application Information
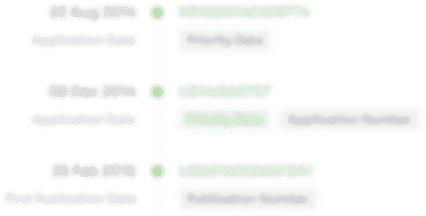
- R&D Engineer
- R&D Manager
- IP Professional
- Industry Leading Data Capabilities
- Powerful AI technology
- Patent DNA Extraction
Browse by: Latest US Patents, China's latest patents, Technical Efficacy Thesaurus, Application Domain, Technology Topic, Popular Technical Reports.
© 2024 PatSnap. All rights reserved.Legal|Privacy policy|Modern Slavery Act Transparency Statement|Sitemap|About US| Contact US: help@patsnap.com