Real scene three-dimensional semantic reconstruction method and device based on deep learning and storage medium
A deep learning and three-dimensional technology, applied in the field of remote sensing surveying and mapping geographic information, can solve the problem of inaccurate labeling of multiple scenes, and achieve the effect of improving computing speed, unaffected network performance, and high-precision segmentation
- Summary
- Abstract
- Description
- Claims
- Application Information
AI Technical Summary
Problems solved by technology
Method used
Image
Examples
Embodiment Construction
[0064] The technical solutions in the embodiments of the present application will be clearly and completely described below in conjunction with the drawings in the embodiments of the present application. Apparently, the described embodiments are some of the embodiments of the present application, but not all of them. Based on the embodiments in this application, all other embodiments obtained by persons of ordinary skill in the art without making creative efforts belong to the scope of protection of this application.
[0065] As a challenging task, semantic 3D modeling has received extensive attention in recent years. With the help of small UAVs, multi-view and high-resolution aerial images of large-scale scenes can be easily collected. This application proposes a real-scene 3D semantic reconstruction method based on deep learning, which obtains the semantic probability distribution of 2D images through a convolutional neural network; utilizes motion recovery structure (SfM, s...
PUM
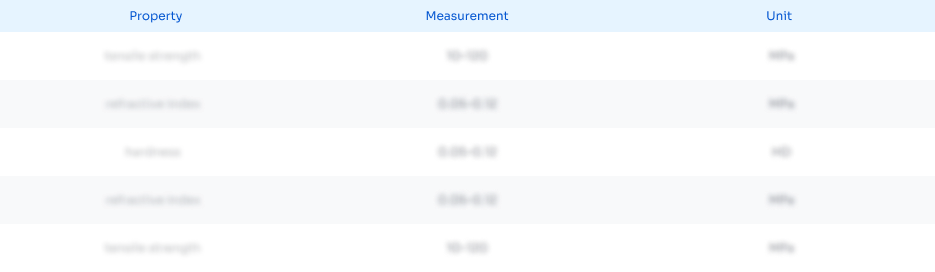
Abstract
Description
Claims
Application Information
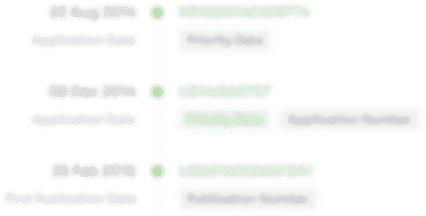
- R&D Engineer
- R&D Manager
- IP Professional
- Industry Leading Data Capabilities
- Powerful AI technology
- Patent DNA Extraction
Browse by: Latest US Patents, China's latest patents, Technical Efficacy Thesaurus, Application Domain, Technology Topic.
© 2024 PatSnap. All rights reserved.Legal|Privacy policy|Modern Slavery Act Transparency Statement|Sitemap