Texture recognition method based on deep self-attention network and local feature coding
A technology of local features and attention, applied in character and pattern recognition, image coding, neural learning methods, etc., can solve problems such as method optimization, limited ability to extract texture features, neglect texture coding, etc., to achieve optimal effectiveness and improve classification. The effect of accuracy
- Summary
- Abstract
- Description
- Claims
- Application Information
AI Technical Summary
Problems solved by technology
Method used
Image
Examples
Embodiment 1
[0050] First, the proposed backbone network based on a deep self-attention network (Transformer) is trained on the ImageNet training dataset to obtain pre-trained weights. Use texture / material related dataset images (DTD, MINC, FMD, Fabrics) to block the image first, see figure 2 , the initial sub-block size is 4*4 pixels, and then the image block is mapped to a dimension of 96 through the feature embedding layer, so that the overall image input dimension is 3136*96; the vector is input to the feature extractor in the PET network. Please see figure 1 The first three window-based self-attention modules (WMSA) 101 of the feature extractor, firstly through the first three window-based self-attention modules (WSMA) of the feature extractor to perform window merging and local self-attention calculation to obtain the feature x 3 , the output dimension is 49*768. Please see figure 1 In the fourth stage of the feature extractor, the global multi-head self-attention module (MSA) 10...
PUM
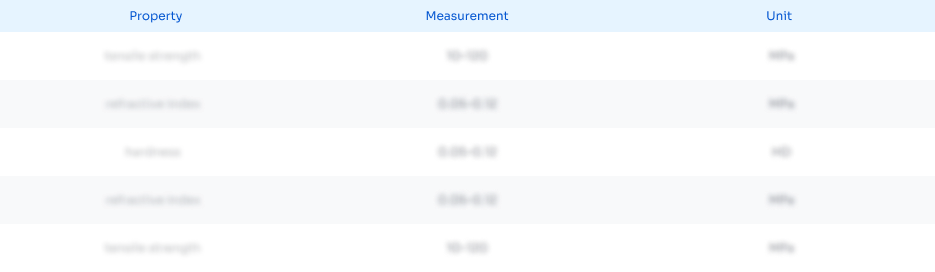
Abstract
Description
Claims
Application Information
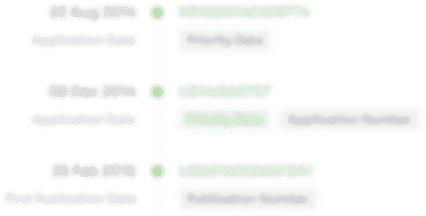
- R&D
- Intellectual Property
- Life Sciences
- Materials
- Tech Scout
- Unparalleled Data Quality
- Higher Quality Content
- 60% Fewer Hallucinations
Browse by: Latest US Patents, China's latest patents, Technical Efficacy Thesaurus, Application Domain, Technology Topic, Popular Technical Reports.
© 2025 PatSnap. All rights reserved.Legal|Privacy policy|Modern Slavery Act Transparency Statement|Sitemap|About US| Contact US: help@patsnap.com