A Privacy Computing Method Based on Heterogeneous Neural Network Model
A neural network model and computing method technology, applied in biological neural network models, neural learning methods, digital data protection, etc., can solve problems such as reducing the accuracy of neural network models, improve training efficiency, promote flow, and expand applications. range effect
- Summary
- Abstract
- Description
- Claims
- Application Information
AI Technical Summary
Problems solved by technology
Method used
Image
Examples
Embodiment 1
[0031] A privacy calculation method based on a heterogeneous neural network model, the heterogeneous neural network model is a neural network model comprising at least one multiplication neuron 15, please refer to the attached figure 1 , the privacy calculation method includes: step A01) establishing a service node and an execution node, several participants participating in the privacy calculation provide an objective function or sample data to the service node, and the service node establishes and trains a heterogeneous neural network model according to the objective function or sample data . Step A02) The service node constructs several sub-models, which correspond to the neurons of the first layer one by one, the input of the sub-model is the neuron of the 0th layer connected to the corresponding neuron of the first layer, and the output of the sub-model is the first layer The input number of neurons, the sub-model corresponding to multiplication neuron 15 is recorded as t...
Embodiment 2
[0053] A privacy calculation method based on a heterogeneous neural network model. On the basis of the first embodiment, this embodiment provides an improved solution method for the heterogeneous neural network model, so that the main model can obtain a confidentiality effect. Please refer to the attached Figure 11 , on the basis of Embodiment 1, the embodiment also includes the following steps: Step F01) The service node generates a confusion coefficient for each multiplication neuron 15, and divides the weight coefficients of the connections involved in the multiplication neuron 15 in the main model by the corresponding Then the main model is sent to the execution node; Step F02) The input neuron of the multiplication sub-model is set with an adjustment coefficient, and the privacy number of the participant is multiplied by the adjustment coefficient and then split into several multipliers ; Step F03) The service node generates the adjustment coefficient of each input neuro...
PUM
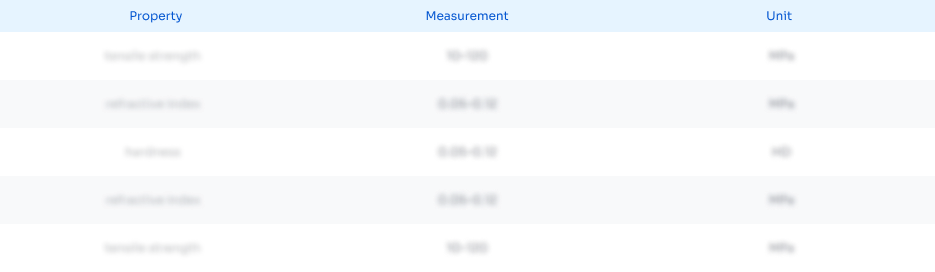
Abstract
Description
Claims
Application Information
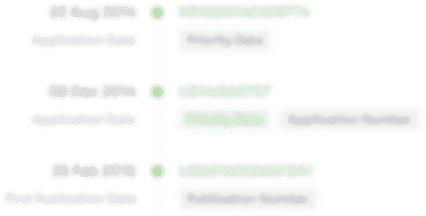
- R&D
- Intellectual Property
- Life Sciences
- Materials
- Tech Scout
- Unparalleled Data Quality
- Higher Quality Content
- 60% Fewer Hallucinations
Browse by: Latest US Patents, China's latest patents, Technical Efficacy Thesaurus, Application Domain, Technology Topic, Popular Technical Reports.
© 2025 PatSnap. All rights reserved.Legal|Privacy policy|Modern Slavery Act Transparency Statement|Sitemap|About US| Contact US: help@patsnap.com