Remote sensing scene image classification method based on multi-level dense feature fusion
A scene image and classification method technology, applied in the field of remote sensing scene image classification, can solve the problem of low classification accuracy of hyperspectral images, and achieve the effect of improving generalization ability and reducing complexity
- Summary
- Abstract
- Description
- Claims
- Application Information
AI Technical Summary
Problems solved by technology
Method used
Image
Examples
specific Embodiment approach 1
[0021] Specific implementation mode 1: In this implementation mode, the remote sensing scene image classification method based on dense fusion of multi-level features The specific process is as follows:
[0022] Step 1, collect hyperspectral image data set X and corresponding label vector data set Y;
[0023] Step 2. Establish a lightweight convolutional neural network BMDF-LCNN based on dense fusion of dual-branch multi-level features;
[0024] Step 3. Input the hyperspectral image dataset X and the corresponding label vector dataset Y into the established lightweight convolutional neural network BMDF-LCNN based on the dense fusion of dual-branch multi-level features, and use the Momentum algorithm for iterative optimization , get the optimal network BMDF-LCNN;
[0025] Step 4: Input the hyperspectral image to be tested into the optimal network BMDF-LCNN to predict the classification result.
specific Embodiment approach 2
[0026] Specific embodiment two: the difference between this embodiment and specific embodiment one is that in said step two, a lightweight convolutional neural network BMDF-LCNN based on the dense fusion of dual-branch multi-level features is established; the specific process is:
[0027] The lightweight convolutional neural network BMDF-LCNN based on the dense fusion of dual-branch multi-level features includes the input layer, the first group Group1, the second group2, the third group3, the fourth group4, the fifth group5, the sixth Group Group6, seventh group Group7, eighth group Group8, ninth group Group9 and output classification layer.
[0028] Other steps and parameters are the same as those in Embodiment 1.
specific Embodiment approach 3
[0029] Specific embodiment three: the difference between this embodiment and specific embodiment one or two is that the connection relationship of the lightweight convolutional neural network BMDF-LCNN based on the dense fusion of dual-branch multi-level features is:
[0030] The output end of the input layer is connected to the first group Group1, the output end of the first group Group1 is connected to the second group Group2, the output end of the second group Group2 is connected to the third group Group3, and the output end of the third group Group3 is connected to the fourth group Group4, The output terminal of the fourth group Group4 is connected to the fifth group Group5, the output terminal of the fifth group Group5 is connected to the sixth group Group6, the output terminal of the sixth group Group6 is connected to the seventh group Group7, and the output terminal of the seventh group Group7 is connected to the eighth group Group8, the output end of the eighth group Gr...
PUM
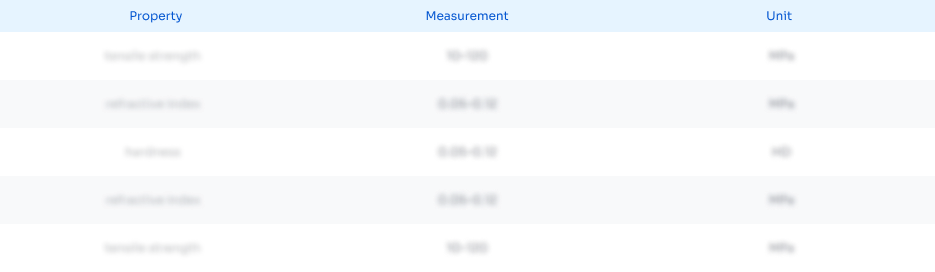
Abstract
Description
Claims
Application Information
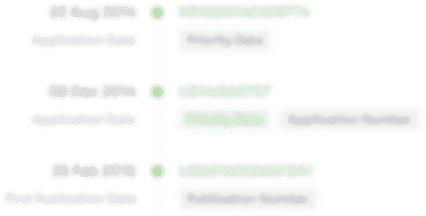
- R&D Engineer
- R&D Manager
- IP Professional
- Industry Leading Data Capabilities
- Powerful AI technology
- Patent DNA Extraction
Browse by: Latest US Patents, China's latest patents, Technical Efficacy Thesaurus, Application Domain, Technology Topic, Popular Technical Reports.
© 2024 PatSnap. All rights reserved.Legal|Privacy policy|Modern Slavery Act Transparency Statement|Sitemap|About US| Contact US: help@patsnap.com