Feature selection method based on covariance metric factor
A feature selection method and measurement factor technology, applied in special data processing applications, instruments, electrical digital data processing, etc., can solve the problems of reducing the classification performance of the classifier, too large space dimension, etc., and achieve the effect of reliable feature selection algorithm.
- Summary
- Abstract
- Description
- Claims
- Application Information
AI Technical Summary
Problems solved by technology
Method used
Image
Examples
Embodiment Construction
[0047] The present invention will be described in detail below in conjunction with the accompanying drawings and specific embodiments.
[0048] The present invention provides a feature selection method based on covariance measurement factors, such as figure 1 As shown, the specific steps are as follows:
[0049] Step 1. Select different text type data sets, and perform preprocessing operations, that is, perform word segmentation operations, and remove stop words in the text. The text data is represented by the vector space model, and the feature words that appear in the data are more than 25% of the total number of documents or less than 3 are removed. The data set is divided according to the ratio of 9:1, that is, 90% of the samples in the data set are randomly selected as the training set data, and the remaining 10% of the samples are used as the test set data.
[0050] Step 2. Set the size of the optimal feature subset to C, use the feature sorting function to calculate t...
PUM
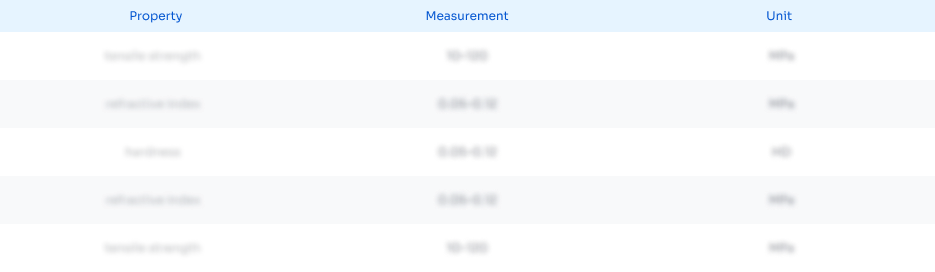
Abstract
Description
Claims
Application Information
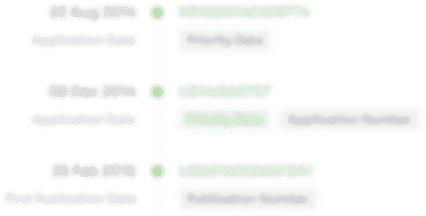
- R&D Engineer
- R&D Manager
- IP Professional
- Industry Leading Data Capabilities
- Powerful AI technology
- Patent DNA Extraction
Browse by: Latest US Patents, China's latest patents, Technical Efficacy Thesaurus, Application Domain, Technology Topic.
© 2024 PatSnap. All rights reserved.Legal|Privacy policy|Modern Slavery Act Transparency Statement|Sitemap