Click rate estimation model based on feature representation under attention mechanism
A prediction model and attention technology, applied in biological neural network models, complex mathematical operations, neural architectures, etc., can solve problems such as inability to predict click rates, limited model learning ability, and dependent prediction effects, etc., to overcome the scale of parameters Restrict, eliminate influence, predict the effect of accurate data
- Summary
- Abstract
- Description
- Claims
- Application Information
AI Technical Summary
Problems solved by technology
Method used
Image
Examples
Embodiment Construction
[0042] In order to make the technical means, creative features, goals and effects achieved by the present invention easy to understand, the following is a detailed description of the click-through rate prediction model based on feature representation under an attention mechanism of the present invention in conjunction with the embodiments and accompanying drawings.
[0043]
[0044] figure 1 It is a structural block diagram of the click rate prediction model based on feature representation under the attention mechanism in the embodiment of the present invention.
[0045] Such as figure 1 As shown, the click rate prediction model 100 based on feature representation under the attention mechanism includes: a feature embedding layer 101 , an explicit feature intersection network 102 , an implicit feature intersection network 103 and an output layer 104 of predicted probability.
[0046] In this embodiment, user-side features, advertisement-side features, and contextual features...
PUM
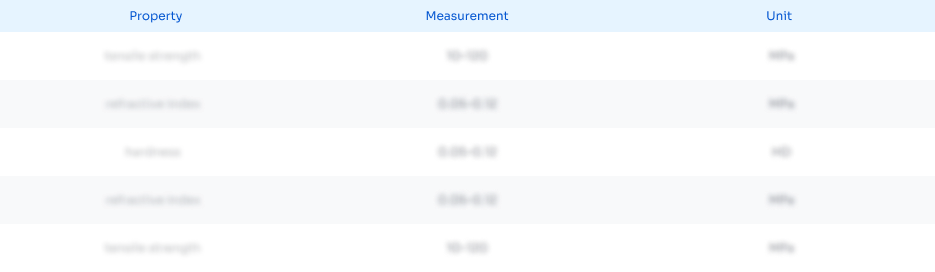
Abstract
Description
Claims
Application Information
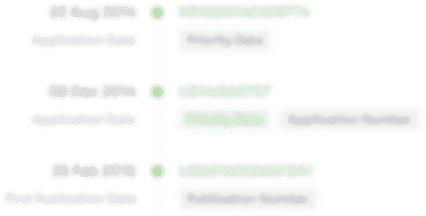
- R&D Engineer
- R&D Manager
- IP Professional
- Industry Leading Data Capabilities
- Powerful AI technology
- Patent DNA Extraction
Browse by: Latest US Patents, China's latest patents, Technical Efficacy Thesaurus, Application Domain, Technology Topic.
© 2024 PatSnap. All rights reserved.Legal|Privacy policy|Modern Slavery Act Transparency Statement|Sitemap