Laser point cloud three-dimensional target detection model and method for complex traffic scene
A traffic scene, laser point cloud technology, applied in three-dimensional object recognition, biological neural network model, character and pattern recognition and other directions, can solve the problem that cannot meet the perception needs of smart cars, cannot adapt to complex traffic scenes, and the detection effect of small objects is not good. It is beneficial to real-time detection, alleviating category imbalance, and making the network easier to optimize.
- Summary
- Abstract
- Description
- Claims
- Application Information
AI Technical Summary
Problems solved by technology
Method used
Image
Examples
Embodiment Construction
[0049] The present invention will be further described below in conjunction with accompanying drawing.
[0050] A laser point cloud three-dimensional target detection method for complex traffic environments proposed by the present invention, such as figure 1 As shown, it specifically includes the following process:
[0051] Step 1. Select the Huawei ONCE data set as the training data set and verification data set of the detection network, and add a class-balanced sampling enhancement method during training.
[0052] The Huawei ONCE dataset is collected by 7 cameras and 1 40-line lidar, which contains 5 categories, namely cars, trucks, buses, pedestrians and cyclists. The data set collects scenes of different weather (sunny, cloudy, rainy), different time (morning, Chinese, afternoon, evening), and different road conditions (city center, suburbs, highways, tunnels, bridges), which can be better Represents complex traffic situations. The ONCE data set collects 144 hours of au...
PUM
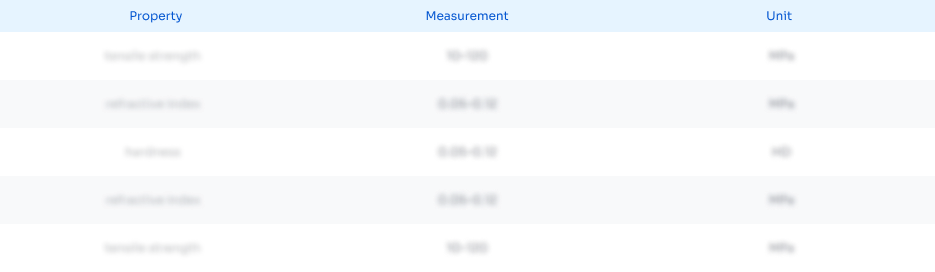
Abstract
Description
Claims
Application Information
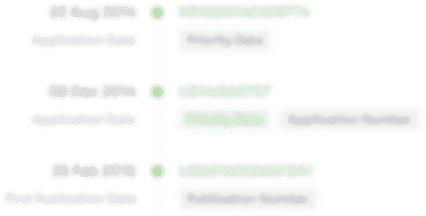
- R&D
- Intellectual Property
- Life Sciences
- Materials
- Tech Scout
- Unparalleled Data Quality
- Higher Quality Content
- 60% Fewer Hallucinations
Browse by: Latest US Patents, China's latest patents, Technical Efficacy Thesaurus, Application Domain, Technology Topic, Popular Technical Reports.
© 2025 PatSnap. All rights reserved.Legal|Privacy policy|Modern Slavery Act Transparency Statement|Sitemap|About US| Contact US: help@patsnap.com