Mechanical arm manipulation skill learning method based on task decomposition
A task decomposition and learning method technology, applied in the field of task decomposition-based manipulator operation skills learning and manipulator complex operation skills learning, can solve problems such as low skill reuse rate, long training time, and unknown effects, and reduce income. The effect of indexing dimension, reducing learning difficulty, improving reuse rate and
- Summary
- Abstract
- Description
- Claims
- Application Information
AI Technical Summary
Problems solved by technology
Method used
Image
Examples
Embodiment Construction
[0028] The present invention will be described in further detail below in conjunction with accompanying drawings and examples.
[0029] The method for learning manipulator operation skills based on task decomposition in the present invention converts complex tasks into multiple subtasks by artificially decomposing some complex tasks, such as stacking blocks, inserting shafts into holes, etc., and then extracts and defines some subtasks from the subtasks. Semantic-level skills or actions, such as "pick", "place", "insert", etc.; then, use imitation learning to learn the underlying skill execution network corresponding to these semantic-level skills ; finally, by means of reinforcement learning, a decision network that can output appropriate semantic-level skills according to the current scene is learned.
[0030] The present invention is based on the method for learning manipulator operation skills of task decomposition. The block diagram of the overall method is as follows: f...
PUM
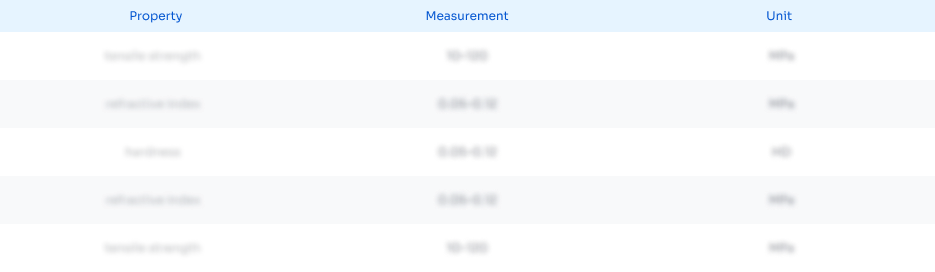
Abstract
Description
Claims
Application Information
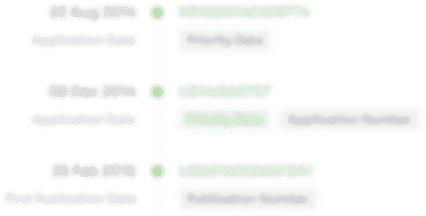
- R&D Engineer
- R&D Manager
- IP Professional
- Industry Leading Data Capabilities
- Powerful AI technology
- Patent DNA Extraction
Browse by: Latest US Patents, China's latest patents, Technical Efficacy Thesaurus, Application Domain, Technology Topic, Popular Technical Reports.
© 2024 PatSnap. All rights reserved.Legal|Privacy policy|Modern Slavery Act Transparency Statement|Sitemap|About US| Contact US: help@patsnap.com