Face image restoration method based on multi-scale local self-attention generative adversarial network
A face image and repair method technology, applied in the direction of biological neural network model, image enhancement, image analysis, etc., can solve the problem of blurring of missing areas in images, achieve stability in the training process, avoid model collapse, enhance expression and The effect of repair efficiency
- Summary
- Abstract
- Description
- Claims
- Application Information
AI Technical Summary
Problems solved by technology
Method used
Image
Examples
Embodiment Construction
[0050] The present invention will be described in detail below in conjunction with the accompanying drawings and embodiments.
[0051] A face image repair method based on multi-scale local self-attention generation confrontation network in this embodiment, which includes:
[0052] Step 1: Obtain the original face image x and the corresponding binary defect mask M; construct a defect face image dataset {x M |x M =M☉x}, and the corresponding original image dataset {x}, preprocess the acquired defective face image dataset, that is, the image size is uniformly set to N 0 ×N 0 , N 0 is the number of pixels of the image in the width dimension and height dimension, N 0 =128, ☉ means that elements are multiplied, and standardized processing is carried out before the input network; the face image data after preprocessing is divided into a training set and a test set according to a ratio of 10:1; in this embodiment, 22,000 different people The face image data set is divided into a ...
PUM
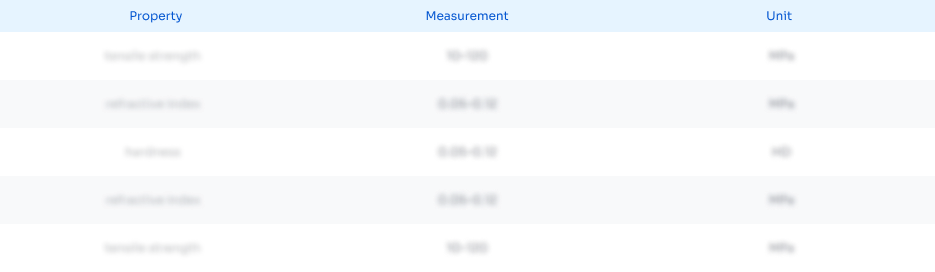
Abstract
Description
Claims
Application Information
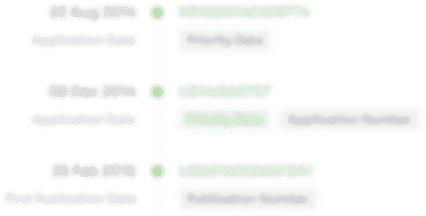
- R&D
- Intellectual Property
- Life Sciences
- Materials
- Tech Scout
- Unparalleled Data Quality
- Higher Quality Content
- 60% Fewer Hallucinations
Browse by: Latest US Patents, China's latest patents, Technical Efficacy Thesaurus, Application Domain, Technology Topic, Popular Technical Reports.
© 2025 PatSnap. All rights reserved.Legal|Privacy policy|Modern Slavery Act Transparency Statement|Sitemap|About US| Contact US: help@patsnap.com