Few-sample target detection method based on singular value decomposition feature enhancement
A singular value decomposition and target detection technology, applied in the field of few-sample target detection based on singular value decomposition feature enhancement, can solve the problems of difficult generalization of features, difficult target classification and positioning, and weak discriminative ability, so as to improve representativeness, Improve generalization and discrimination, and improve the effect of positioning and classification accuracy
- Summary
- Abstract
- Description
- Claims
- Application Information
AI Technical Summary
Problems solved by technology
Method used
Image
Examples
Embodiment 1
[0039] In the existing few-sample target detection methods, due to the small number of samples, the extracted features cannot well represent the features of this category, and the learned model has poor generalization and discrimination. The present invention conducts research on the above problems, and provides a few-sample target detection method based on singular value decomposition feature enhancement.
[0040] The present invention is a few-sample target detection method based on singular value decomposition feature enhancement, see figure 1 , figure 1 It is a flow chart of the present invention, and the few-sample target detection method based on singular value decomposition feature enhancement of the present invention comprises the following steps:
[0041] (1) Obtain the image data set for target detection: the image data set includes a training sample set and a test sample set. The commonly used data sets for few-sample target detection are PASCAL VOC and COCO data s...
Embodiment 2
[0068] The few-sample target detection method based on singular value decomposition feature enhancement is the same as embodiment 1, and the construction feature enhancement module described in step (3) carries out feature enhancement and includes the following steps:
[0069] 3.1) Singular value decomposition is performed on the feature map: the feature map F extracted in step (2), rescaled to Singular value decomposition is performed on the adjusted feature map F to obtain where U is the left singular matrix after singular value decomposition, V is the right singular matrix after singular value decomposition, U and V are orthogonal unitary matrices, Σ is an m×n diagonal matrix sorted by size of the singular values of the diagonal, its dimension is 2k, m is the number of channels of the feature map F, and ω is the width of the feature map F , h is the height of the feature map F, n is the dimension of the adjusted feature map F, n=ω×h.
[0070] 3.2) Learning genera...
Embodiment 3
[0080] The few-sample target detection method based on singular value decomposition feature enhancement is the same as embodiment 1-2, two kinds of feature maps described in step (6) are fused to form a feature fusion layer, including the following steps:
[0081] 6.1) Generalized feature map fusion of advanced discriminant information: The generated feature map P of the candidate frame area is coded and represented by the codeword set learned by dictionary learning, expressed as Rep, and then the representation Rep is combined with the generated feature map P of the candidate frame area Alignment is performed through the RoI alignment layer. After alignment, the two are merged to achieve feature fusion through matrix splicing, and a generalized feature map [φ(P),φ(Rep)] with fusion features is obtained. The representation process is as follows:
[0082]
[0083] Among them, ψ is a fully connected layer that maps the feature map P to the dictionary space, p is the feature su...
PUM
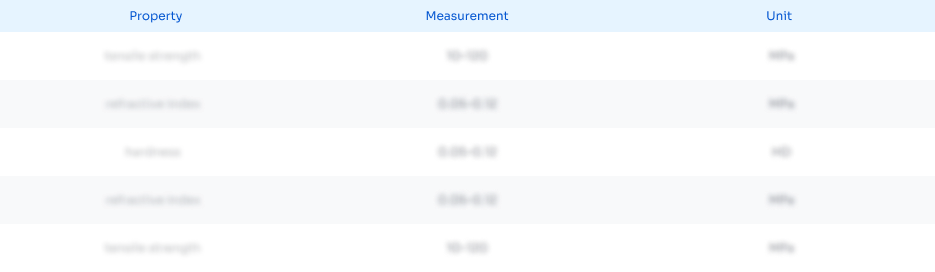
Abstract
Description
Claims
Application Information
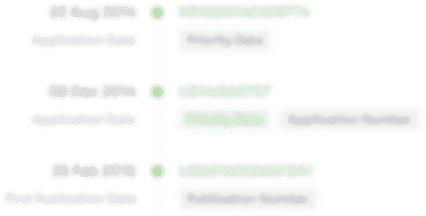
- R&D Engineer
- R&D Manager
- IP Professional
- Industry Leading Data Capabilities
- Powerful AI technology
- Patent DNA Extraction
Browse by: Latest US Patents, China's latest patents, Technical Efficacy Thesaurus, Application Domain, Technology Topic, Popular Technical Reports.
© 2024 PatSnap. All rights reserved.Legal|Privacy policy|Modern Slavery Act Transparency Statement|Sitemap|About US| Contact US: help@patsnap.com