Battery defect detection method based on lightweight neural network
A technology of defect detection and neural network, applied in the direction of neural learning method, biological neural network model, neural architecture, etc., can solve the problems of limited target detection model application, difficult implementation of battery defect, high system cost, etc., to reduce quality inspection Process, reduce artificial quality in the later stage, suppress the effect of the background
- Summary
- Abstract
- Description
- Claims
- Application Information
AI Technical Summary
Problems solved by technology
Method used
Image
Examples
Embodiment Construction
[0022] The technical solutions of the present invention will be clearly and completely described below in conjunction with the accompanying drawings, but this does not limit the protection scope of the present application.
[0023] The present invention provides a battery defect detection method (abbreviated method) based on a lightweight neural network, comprising the following steps:
[0024] The first step is to collect images;
[0025] Use industrial cameras to collect battery images as the original images for defect detection; original images include images without defects and images containing defects to be detected; images containing defects to be detected can be images containing a single defect or multiple defects The image must contain all types of defects to be detected.
[0026] The second step is to perform prediction processing on the image to obtain a data set;
[0027] 2-1. Create a data set storage folder
[0028] Create a new VOCdevkit folder, VOC2007 fold...
PUM
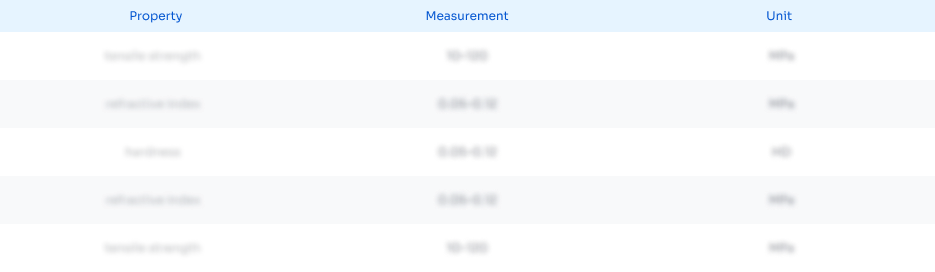
Abstract
Description
Claims
Application Information
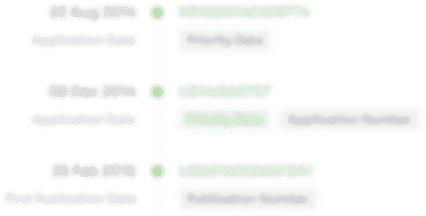
- R&D Engineer
- R&D Manager
- IP Professional
- Industry Leading Data Capabilities
- Powerful AI technology
- Patent DNA Extraction
Browse by: Latest US Patents, China's latest patents, Technical Efficacy Thesaurus, Application Domain, Technology Topic, Popular Technical Reports.
© 2024 PatSnap. All rights reserved.Legal|Privacy policy|Modern Slavery Act Transparency Statement|Sitemap|About US| Contact US: help@patsnap.com