Wireless sensor node fault diagnosis method and device based on deep learning
A wireless sensor and deep learning technology, applied in the field of fault diagnosis of wireless sensor nodes based on deep learning, can solve problems such as fusion error faults and stuck faults, achieve enhanced robustness and applicability, and facilitate continuous iterative improvement and update , the effect of strong model generalization ability
- Summary
- Abstract
- Description
- Claims
- Application Information
AI Technical Summary
Problems solved by technology
Method used
Image
Examples
Embodiment 1
[0055] This embodiment proposes a wireless sensor node fault diagnosis method based on deep learning for the complex environment of the nuclear power plant, which improves the reliability of the wireless sensor network nodes and the entire network and the safety of each operating equipment of the nuclear power plant.
[0056] In this embodiment, through in-depth research and analysis of the fault model of the wireless sensor node mechanism, combined with the experimental fault data, the source domain training set data is obtained. The present invention makes full use of the deep essential feature extraction ability of SSDAE and the robustness and adaptability of the model, and obtains a deep fault feature extractor through training on the source domain data set; a fully connected adaptive layer is added after the SSDAE deep feature extractor to achieve A small amount of actual fault sample data in the target domain is used to train and correct the migration model, which can mak...
Embodiment 2
[0134] This embodiment proposes a wireless sensor node fault diagnosis device based on deep learning, such as Figure 7 As shown, the device includes a data preprocessing module, a training module, a migration module, an optimization module, and a detection module.
[0135] Wherein, the data preprocessing module is used to obtain SSDAE model source domain training set data according to wireless sensor node mechanism failure model data and experimental data.
[0136] The training module obtains the SSDAE model by training on the source domain training set data.
[0137] The migration module migrates the trained SSDAE model to obtain a migration model, trains and corrects the migration model with fault sample data in the target domain, and obtains the final deep fault diagnosis model.
[0138] The optimization module identifies the fault categories that cannot be judged by the deep fault diagnosis model by adding the "Don't know" fault response label to the classification layer...
PUM
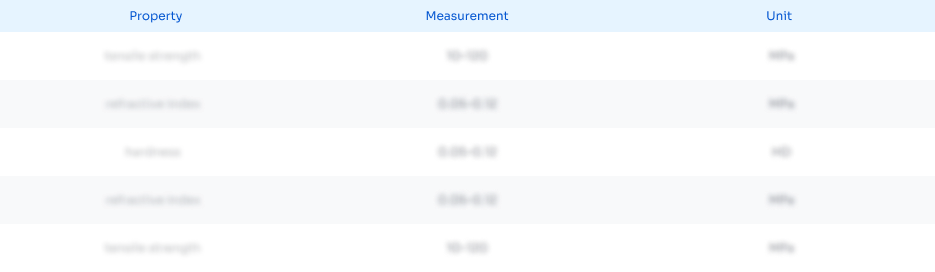
Abstract
Description
Claims
Application Information
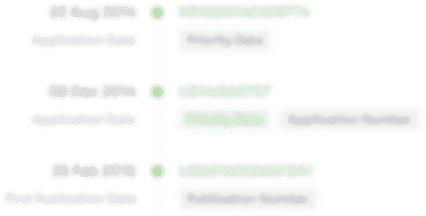
- R&D Engineer
- R&D Manager
- IP Professional
- Industry Leading Data Capabilities
- Powerful AI technology
- Patent DNA Extraction
Browse by: Latest US Patents, China's latest patents, Technical Efficacy Thesaurus, Application Domain, Technology Topic, Popular Technical Reports.
© 2024 PatSnap. All rights reserved.Legal|Privacy policy|Modern Slavery Act Transparency Statement|Sitemap|About US| Contact US: help@patsnap.com