Aero-engine fault diagnosis method based on transferable neural network
An aero-engine and fault diagnosis technology, applied in neural learning methods, biological neural network models, neural architectures, etc., can solve the problems of not landing, staying, unrealistic, etc., to reduce distribution differences and improve fault diagnosis accuracy. Effect
- Summary
- Abstract
- Description
- Claims
- Application Information
AI Technical Summary
Problems solved by technology
Method used
Image
Examples
Embodiment Construction
[0079] In the case of multi-fault diagnosis of aeroengines, firstly, the objective function of the original RVFL model is established based on all collected engine operating data (including normal data sample information and fault data sample information)
[0080]
[0081] in is the sample, N is the total number of samples, and d is the number of features. For the i-th instance, x i is a d-dimensional feature vector. is the output weight, is the jth row of β. w j and b j The weights and biases of the input to the enhancement layer are randomly set. T=[t i ,...t N ] T is the label set of the sample, if x i belongs to class j, then t ij is 1, and the rest are 0. h j (·) is the activation function of the jth enhanced node, for x i , which is defined as:
[0082]
[0083] Furthermore, formula (1) can be further expressed as a matrix, and its objective function is:
[0084]
[0085] where matrix The definition of is shown in the following formula
[0...
PUM
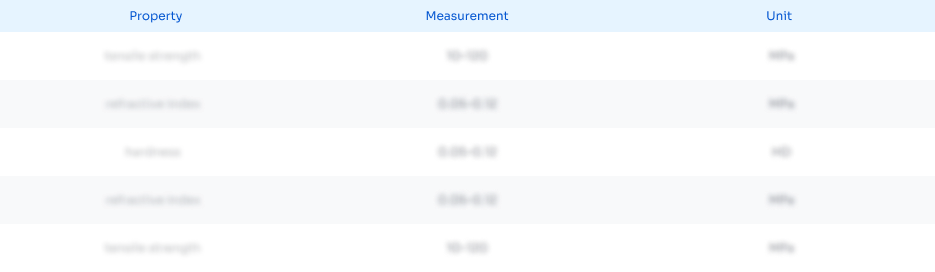
Abstract
Description
Claims
Application Information
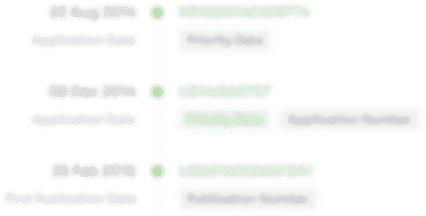
- R&D
- Intellectual Property
- Life Sciences
- Materials
- Tech Scout
- Unparalleled Data Quality
- Higher Quality Content
- 60% Fewer Hallucinations
Browse by: Latest US Patents, China's latest patents, Technical Efficacy Thesaurus, Application Domain, Technology Topic, Popular Technical Reports.
© 2025 PatSnap. All rights reserved.Legal|Privacy policy|Modern Slavery Act Transparency Statement|Sitemap|About US| Contact US: help@patsnap.com