Neural network model quantification method, device and system, electronic equipment and storage medium
A neural network model and quantification method technology, applied in electronic equipment and storage media, devices and systems, neural network model quantization method field, can solve problems such as quantization error, increase hardware design overhead, neural network prediction performance loss, etc. The effect of improving accuracy, reducing distribution differences, and reducing quantization errors
- Summary
- Abstract
- Description
- Claims
- Application Information
AI Technical Summary
Problems solved by technology
Method used
Image
Examples
Embodiment Construction
[0038] Various exemplary embodiments of the present disclosure will now be described in detail with reference to the accompanying drawings. It should be noted that relative arrangements of components and steps, numerical expressions and numerical values set forth in these embodiments do not limit the scope of the present disclosure unless specifically stated otherwise.
[0039] Those skilled in the art can understand that terms such as "first" and "second" in the embodiments of the present disclosure are only used to distinguish different steps, devices or modules, etc. necessary logical sequence.
[0040] It should also be understood that in the embodiments of the present disclosure, "plurality" may refer to two or more than two, and "at least one" may refer to one, two or more than two.
[0041] It should also be understood that any component, data or structure mentioned in the embodiments of the present disclosure can generally be understood as one or more unless there i...
PUM
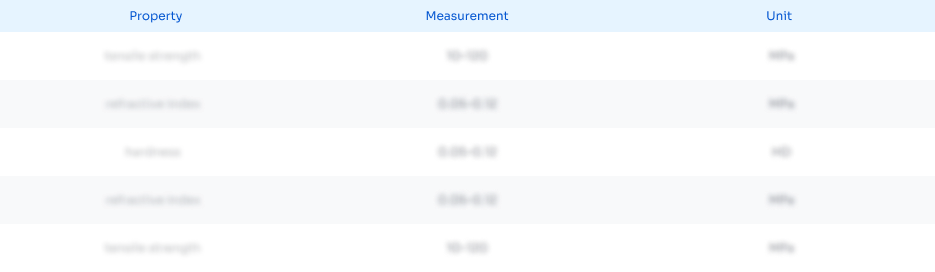
Abstract
Description
Claims
Application Information
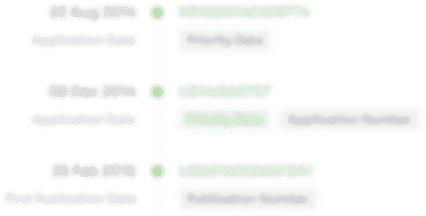
- R&D Engineer
- R&D Manager
- IP Professional
- Industry Leading Data Capabilities
- Powerful AI technology
- Patent DNA Extraction
Browse by: Latest US Patents, China's latest patents, Technical Efficacy Thesaurus, Application Domain, Technology Topic, Popular Technical Reports.
© 2024 PatSnap. All rights reserved.Legal|Privacy policy|Modern Slavery Act Transparency Statement|Sitemap|About US| Contact US: help@patsnap.com