Bearing composite fault diagnosis method based on multi-label field adaptive model
An adaptive model and composite fault technology, applied in the fields of mechanical fault diagnosis, artificial intelligence and signal processing, can solve the problems of model effect decline, ignoring potential relationships, multiple problems, etc., to achieve good robustness and improve the effect of diagnosis accuracy.
- Summary
- Abstract
- Description
- Claims
- Application Information
AI Technical Summary
Problems solved by technology
Method used
Image
Examples
Embodiment
[0082] Using the present invention to diagnose the bearing fault data collected by the self-made bearing fault experiment platform in the laboratory, the fault signal is as follows: figure 2 As shown, the bearing model used in the experiment is 6205 deep groove ball bearing, and the sampling frequency is 10kHz.
[0083] The collected bearing vibration signals under four different motor loads (0kN, 1kN, 2kN, 3kN) are constructed into four data sets C1, C2, C3, and C4. Each load includes 8 types of bearings with different health conditions, and the specific settings are shown in Table 1. They are one normal state (Normal), three single faults (IF, OF, BF) and four compound faults (IB, IO, OB, IOB), and the fault size is 0.2mm.
[0084] Table 1 Composite fault diagnosis dataset settings
[0085]
[0086] Using different data sets as source domain and target domain respectively, multiple groups of diagnostic experiments are carried out using the method of the present inventi...
PUM
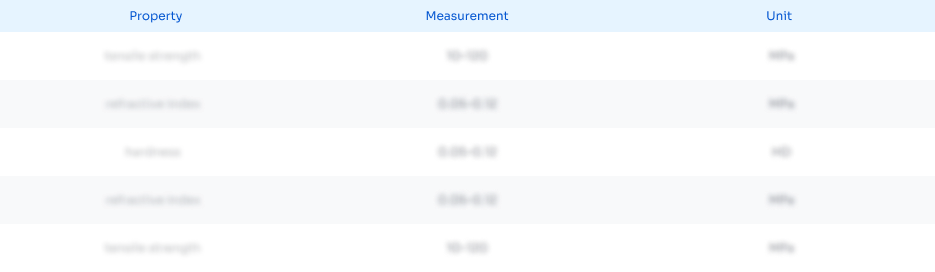
Abstract
Description
Claims
Application Information
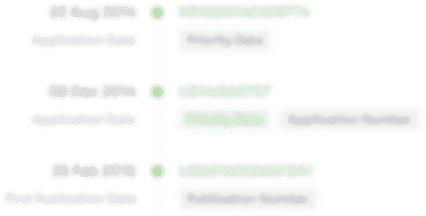
- R&D
- Intellectual Property
- Life Sciences
- Materials
- Tech Scout
- Unparalleled Data Quality
- Higher Quality Content
- 60% Fewer Hallucinations
Browse by: Latest US Patents, China's latest patents, Technical Efficacy Thesaurus, Application Domain, Technology Topic, Popular Technical Reports.
© 2025 PatSnap. All rights reserved.Legal|Privacy policy|Modern Slavery Act Transparency Statement|Sitemap|About US| Contact US: help@patsnap.com