Automatic driving method based on conditional imitation learning and reinforcement learning
A technology of reinforcement learning and automatic driving, applied in neural learning methods, computer components, structured data retrieval, etc., can solve problems such as low efficiency of reinforcement learning exploration, rough rough PP angle, poor interpretability, etc. Achieve the effects of reducing the dependence on training data sets, enhancing robustness, and improving performance
- Summary
- Abstract
- Description
- Claims
- Application Information
AI Technical Summary
Problems solved by technology
Method used
Image
Examples
Embodiment 1
[0053] According to an automatic driving method based on conditional imitation learning and reinforcement learning provided by the present invention, such as Figure 1-Figure 7 shown, including:
[0054] Step S1: Collect driving data, and mark the corresponding decision-making actions as expert decisions;
[0055] Step S2: According to the driving data, calculate the heading angle, and obtain the feature vector and feature map;
[0056] Step S3: Construct a training data set based on the feature vector, feature map, heading angle and expert decision, and use the training data set to train the model;
[0057] Step S4: Apply the trained model to the vehicle to realize the automatic driving decision of the vehicle.
[0058] Specifically, in the step S1:
[0059] The driving data includes vehicle status information and perception information obtained from on-board sensors;
[0060] The vehicle status information includes vehicle position information, speed information, steerin...
Embodiment 2
[0080] Embodiment 2 is a preferred example of Embodiment 1 to describe the present invention more specifically.
PUM
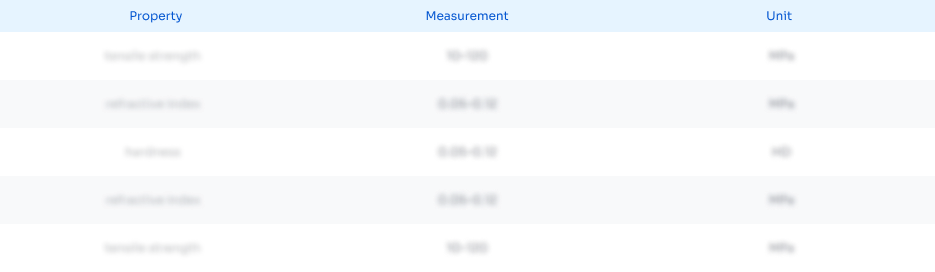
Abstract
Description
Claims
Application Information
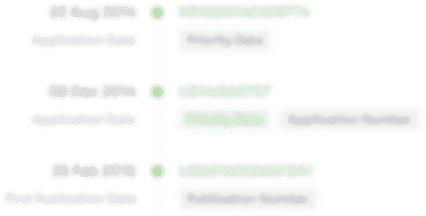
- Generate Ideas
- Intellectual Property
- Life Sciences
- Materials
- Tech Scout
- Unparalleled Data Quality
- Higher Quality Content
- 60% Fewer Hallucinations
Browse by: Latest US Patents, China's latest patents, Technical Efficacy Thesaurus, Application Domain, Technology Topic, Popular Technical Reports.
© 2025 PatSnap. All rights reserved.Legal|Privacy policy|Modern Slavery Act Transparency Statement|Sitemap|About US| Contact US: help@patsnap.com