Clustering-based confrontation partial domain adaptive cross-subject EEG emotion recognition method
An emotion recognition and clustering technology, applied in the field of EEG emotion recognition, can solve problems such as category imbalance and uneven sample distribution, and achieve the effect of improving time efficiency, solving individual differences, and strengthening generalization ability.
- Summary
- Abstract
- Description
- Claims
- Application Information
AI Technical Summary
Problems solved by technology
Method used
Image
Examples
Embodiment Construction
[0039] The present invention will be further described below in conjunction with accompanying drawing.
[0040] Such as figure 1 As shown, it is a model structure diagram of the cross-subject EEG emotion recognition method based on clustering against partial domain adaptation, which mainly includes the following steps:
[0041] Step 1: Data Preprocessing
[0042] The EEG signals of the dataset are preprocessed before being input into the framework. Differential entropy (DE) features were extracted every second from 5 frequency bands of the SEED dataset: δ: 1-3Hz, θ: 4-7Hz, α: 8-13Hz, β: 14-30Hz, γ: 31-50Hz. The feature dimension is 310 (62 channels × 5 frequency bands).
[0043] For a certain length, the approximation follows a Gaussian distribution The EEG signal of , its differential entropy is:
[0044]
[0045] Equal to the logarithm of its energy spectrum in a specific frequency band.
[0046] The SEED dataset is a public dataset from the BCMI Laboratory of Shan...
PUM
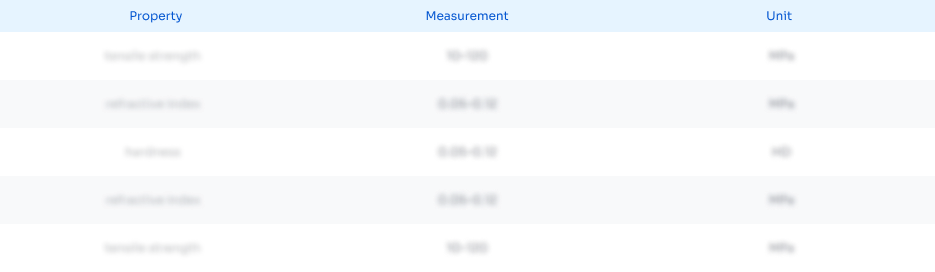
Abstract
Description
Claims
Application Information
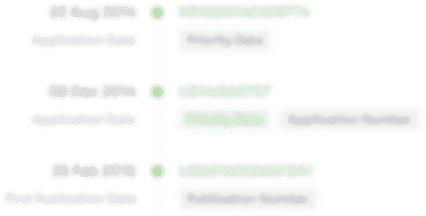
- R&D Engineer
- R&D Manager
- IP Professional
- Industry Leading Data Capabilities
- Powerful AI technology
- Patent DNA Extraction
Browse by: Latest US Patents, China's latest patents, Technical Efficacy Thesaurus, Application Domain, Technology Topic, Popular Technical Reports.
© 2024 PatSnap. All rights reserved.Legal|Privacy policy|Modern Slavery Act Transparency Statement|Sitemap|About US| Contact US: help@patsnap.com