Generation method of teenager suicide risk prediction model and prediction system
A technology for risk prediction and teenagers, applied in computing models, medical data mining, instruments, etc., can solve problems such as low sensitivity, low positive predictive value, and few applications
- Summary
- Abstract
- Description
- Claims
- Application Information
AI Technical Summary
Problems solved by technology
Method used
Image
Examples
Embodiment Construction
[0011] Extreme Gradient Boosting (XGBoost) is a machine learning technique that is an efficient, flexible, and optimized distributed gradient boosting library. In the current study, we used the XGBoost algorithm to train a suicidal behavior detection model and used it to predict possible future suicidal behaviors. We randomly split the dataset into training set and testing set, where the proportions of training set and testing set are 80% and 20%, respectively, and an independent testing dataset is used for model validation. The independent input variables for each person are depression, anxiety, gender, age, BMI, psychological resilience, place of residence, parental relationship, left-behind children, parental expectations, only child, homework, living in school, school violence, and domestic violence as risk factors ; the output variables are suicide attempts and suicide attempts. In addition, we also used Gaussian Naive Bayes (Gaussian Naive Bayes, GNB), Support Vector Ma...
PUM
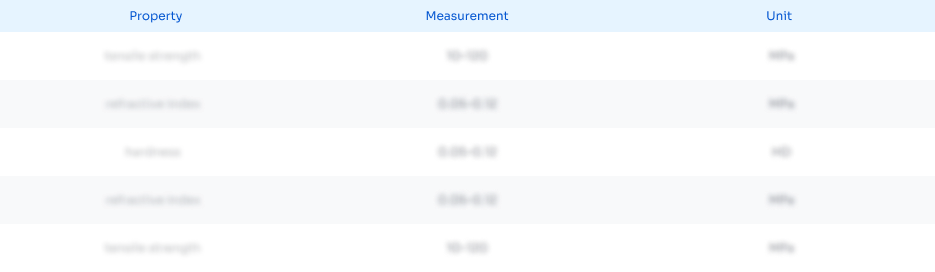
Abstract
Description
Claims
Application Information
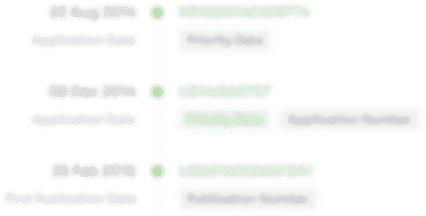
- R&D Engineer
- R&D Manager
- IP Professional
- Industry Leading Data Capabilities
- Powerful AI technology
- Patent DNA Extraction
Browse by: Latest US Patents, China's latest patents, Technical Efficacy Thesaurus, Application Domain, Technology Topic, Popular Technical Reports.
© 2024 PatSnap. All rights reserved.Legal|Privacy policy|Modern Slavery Act Transparency Statement|Sitemap|About US| Contact US: help@patsnap.com