Monte Carlo rendering graph denoising method based on generative adversarial network
A rendering image and network technology, applied in the field of image processing, can solve the problems of long network reasoning time and low image denoising efficiency, and achieve the effects of improving production efficiency, good denoising effect, and helping denoising
- Summary
- Abstract
- Description
- Claims
- Application Information
AI Technical Summary
Problems solved by technology
Method used
Image
Examples
Embodiment
[0063] The denoising method of Monte Carlo rendering image based on generative confrontation network in this embodiment includes: A, training the Monte Carlo rendering image denoising model; B, using the trained Monte Carlo rendering image denoising model to treat denoising The Monte Carlo rendering image is denoised to obtain the denoising result.
[0064] Specifically, in the above step A, the training Monte Carlo rendering image denoising model includes the following sub-steps:
[0065] A1. Establish training data set:
[0066] Use the renderer to render the pre-defined 3D scene with a low sampling rate, obtain the noise rendering image and auxiliary cache image, perform high-sampling rendering on the pre-defined 3D scene, obtain the target rendering image, and use the target rendering image as the training target.
[0067] In order to further increase the denoising performance of the network model, while rendering the noise rendering image with a low number of samples per...
PUM
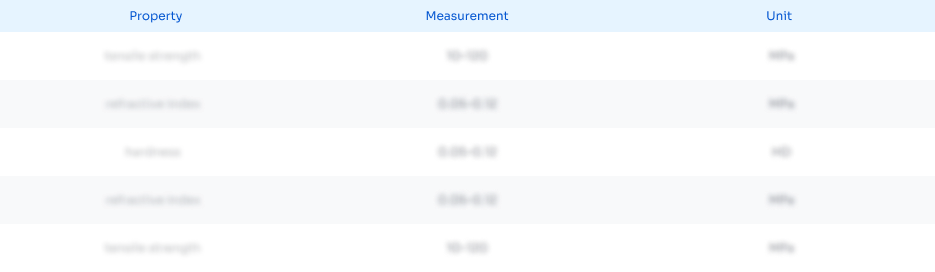
Abstract
Description
Claims
Application Information
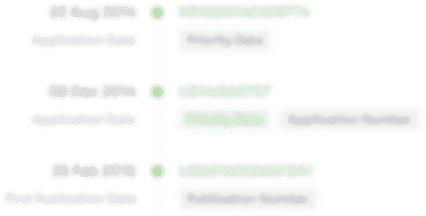
- R&D
- Intellectual Property
- Life Sciences
- Materials
- Tech Scout
- Unparalleled Data Quality
- Higher Quality Content
- 60% Fewer Hallucinations
Browse by: Latest US Patents, China's latest patents, Technical Efficacy Thesaurus, Application Domain, Technology Topic, Popular Technical Reports.
© 2025 PatSnap. All rights reserved.Legal|Privacy policy|Modern Slavery Act Transparency Statement|Sitemap|About US| Contact US: help@patsnap.com