Intelligent prediction method for small molecule-protein binding affinity
An intelligent prediction, protein technology, applied in the analysis of two-dimensional or three-dimensional molecular structure, neural learning methods, instruments, etc., can solve the problems of easily overlooked proteins, the difficulty of mining spatial structure information, etc., and achieve rapid and accurate data processing. Effects of Small Molecule-Protein Affinity Prediction
- Summary
- Abstract
- Description
- Claims
- Application Information
AI Technical Summary
Problems solved by technology
Method used
Image
Examples
Embodiment example 1
[0036] The overall structural framework of the invention is as figure 1 As shown, the input is the SMILES representation of protein sequence and small molecule respectively. First, the small molecule graph is constructed based on SMILES, and the protein weight graph is constructed based on the sequence. Secondly, two graph convolutional neural networks are used to extract the features of molecular graph and protein weight graph respectively. Finally, based on the extracted two vectors, binding affinity prediction is performed.
[0037] (1) Construction of protein weight graph
[0038] Sequences are represented by character strings. In order to fully describe sequence features, the present invention proposes a method for constructing protein weight graphs based on residue interactions. The construction process is as follows: figure 2 shown.
[0039] First, the ESM model is used to predict the interaction of protein residues. This model does not require sequence alignment, a...
Embodiment example 2
[0055] In addition, the method of the present invention and existing method performance comparison (Davis database), the result is as follows:
[0056] Method Protein rep. Compound rep. MSE CI Pearson KronRLS Smith-Waterman Pubchem Sim 0.379 0.871 - SimBoost Smith-Waterman Pubchem Sim 0.282 0.872 - Deep DTA Smith-Waterman Pubchem Sim 0.608 0.790 - Deep DTA Smith-Waterman CNN 0.420 0.886 - Deep DTA CNN Pubchem Sim 0.419 0.835 - Deep DTA CNN CNN 0.261 0.878 - Wide DTA CNN CNN 0.262 0.886 0.820 GraphDTA CNN GIN 0.229 0.893 - GANs DTA GAN GAN 0.276 0.881 - The method of the invention GCN GCN 0.208 0.902 0.862
[0057] The results show that the present invention realizes more accurate small molecule-protein affinity prediction.
PUM
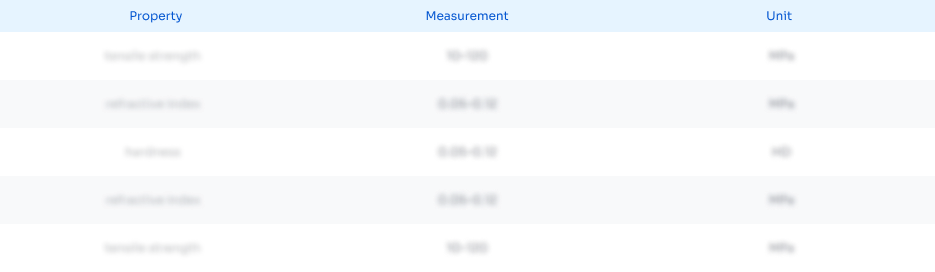
Abstract
Description
Claims
Application Information
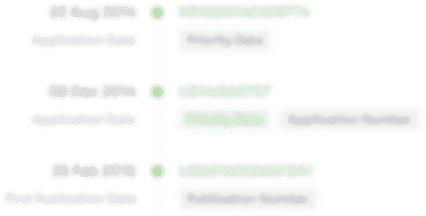
- R&D Engineer
- R&D Manager
- IP Professional
- Industry Leading Data Capabilities
- Powerful AI technology
- Patent DNA Extraction
Browse by: Latest US Patents, China's latest patents, Technical Efficacy Thesaurus, Application Domain, Technology Topic, Popular Technical Reports.
© 2024 PatSnap. All rights reserved.Legal|Privacy policy|Modern Slavery Act Transparency Statement|Sitemap|About US| Contact US: help@patsnap.com