Adaptive signal control method and system based on reinforcement learning and phase competition
An adaptive signal and reinforcement learning technology, which is applied in the traffic control system of road vehicles, traffic control systems, neural learning methods, etc., can solve problems such as poor generalization, discounted control effects, and model no longer applicable, and achieve good Lu Effects of stickiness, reduced state space, and improved convergence
- Summary
- Abstract
- Description
- Claims
- Application Information
AI Technical Summary
Problems solved by technology
Method used
Image
Examples
Embodiment
[0108] The effect of the present invention after training is compared with the traditional signal control method MaxPressure, the reinforcement learning method DQN under ordinary state representation, and the baseline method FRAP from the average travel time, average waiting time, and average queue length. Among them, FRAP is the main reference of the present invention, and the present invention optimizes the structure on the basis of it. The results are shown in Table 1. It can be seen that the present invention has achieved optimal control effects on the three indicators.
[0109] Table 1. Comparison of experimental effects
[0110]
[0111] For the test of generalization performance, the model trained on one data set is directly tested on the other three data sets, and the average travel time of the test results is shown in Table 2. It can be seen that compared with the baseline method FRAP, the present invention achieves lower average travel time on the three test dat...
PUM
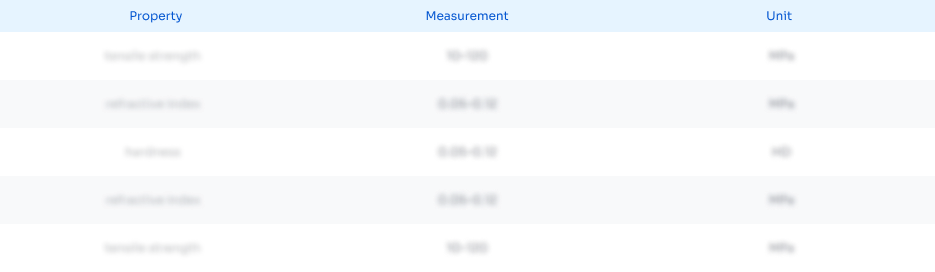
Abstract
Description
Claims
Application Information
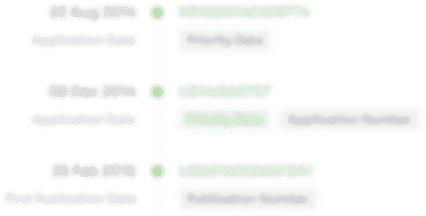
- R&D Engineer
- R&D Manager
- IP Professional
- Industry Leading Data Capabilities
- Powerful AI technology
- Patent DNA Extraction
Browse by: Latest US Patents, China's latest patents, Technical Efficacy Thesaurus, Application Domain, Technology Topic, Popular Technical Reports.
© 2024 PatSnap. All rights reserved.Legal|Privacy policy|Modern Slavery Act Transparency Statement|Sitemap|About US| Contact US: help@patsnap.com